All published articles of this journal are available on ScienceDirect.
Coronavirus: Economic Prospects, Healthcare Policies and a Statistical Model for Italy
In the context of coronavirus, declared as a global pandemic by the World Health Organization [1] on March 11th 2020, to date, Italy has been the western country with the highest number of infections in the early stages [2] and, to date, took drastic measures to slow down the spread of the virus. Due to being the second highest average age of the world's population, the virus has a 6.6% mortality, one of the highest in the world [3]. In Italy, a country with over 60 million inhabitants, there are only approximately 5,200 beds in intensive care and an exponential increase in the number of people infected risks bringing the national health system to collapse [4].
Since January 30th, when all flights to and from China were suspended, a series of measures have been taken to monitor and contain the spread of the virus in Italy. On February 23rd, following the outbreaks in Lombardy and Veneto, a decree law was introduced announcing urgent measures to contain and manage the epidemiological emergency.
This decree was implemented in the following days by further orders of the President of the Council of Ministers (DPCM), including that of March 4th, declaring the suspension of educational activities throughout the country, and on March 8th introducing quarantine measures for an area including whole Lombardy and 14 other provinces, and that of March 9th extending quarantine to the rest of the country (Tables 1 and 2).
The main measures adopted to date can be summarized in the following four points:
• Closure of schools, churches and businesses (with the exception of grocery stores and pharmacies)
• Severe limitations on travel (it is allowed to go out only to go to work, buy basic necessities, or for emergencies)
• Prohibition of assembly in public places
• Invitation to keep a distance of at least one meter from other people
To analyze the trend in Italy, a non-linear regression model has been developed for this research, trying to forecast the number of infected people day by day. It has a very good fit for days 8 to 22 (the last day available for the development of the model, coinciding with March 12th). The equation, where x represents the day and y the total number of forecasted infected patients, with approximated constants is:
![]() |
The model is straightforward and has been obtained with R software, by feeding it with daily observed data ranging from February 20th to March 13th, 2020. Two statistical scenarios on the possible diffusion of COVID-19 in Italy are presented here. In both of them, values for days 24 to 40 are the forecasted ones (ranging from March 14th to March 30th). The first model Fig. (1) is based on the pure non-linear regression equation underlined above. It does not take into account the restrictive measures adopted in Italy and therefore, it should not happen in reality, but it is useful to show what could potentially happen if no containment measures were implemented. There is a basic exponential trend, with a moderate contraction over time, which would lead to about 100,000 cases diagnosed within the thirty-fifth day from the beginning of the epidemic (i.e. March 25th, 2020).
The second model Fig. (2), on the other hand, uses the same non-linear regression function till day 25 (March, 15th), while from day 26 (March, 16th) onwards it takes into account the day/day data directly derived from the data observed in China, following the implementation of the restrictive measures adopted (it should be noted that, even in China, before that point the infection had followed a trend well described by the non-linear regression model developed for this article). The correction has been done by considering day/day real data from China and transposing this ratio to the numbers provided by the NlR model. According to this second model, as of March 25th, the total number of cases diagnosed in Italy should be about 55,000, with a clear trend of progressive attenuation that would lead to a flattening of the curve after another 5 days (61,000 cases diagnosed as of March 30th).
The bend in the curve can be shifted to the right if the restrictive measures do not deliver the expected results by the day foreseen in this model (resulting in an overall increase in the number of cases). However, the general trend should remain the same, albeit with an overall higher number of cases. Hence, the one depicted in Fig. (2) has to be considered an optimistic scenario, but it is being followed very precisely for 24-27 days.
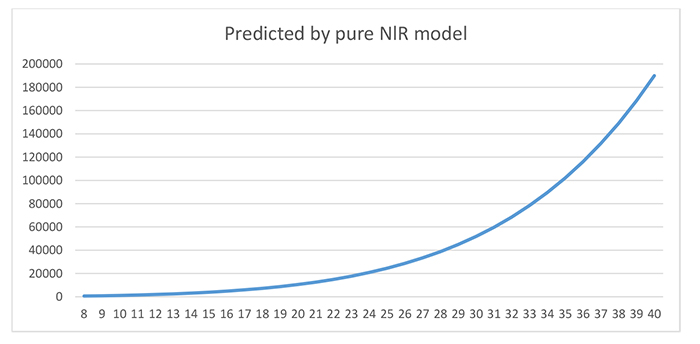
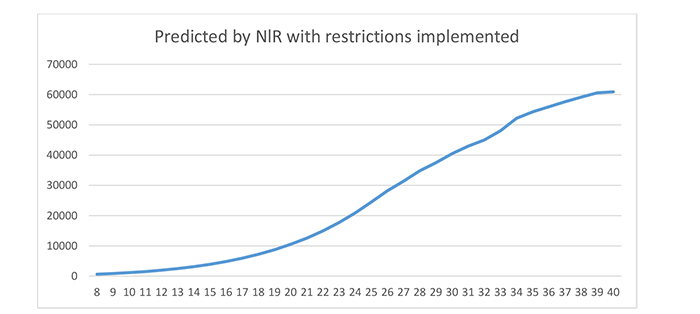
Day # | Observed | Predicted | Error % | Day/Day O | Day/Day P |
---|---|---|---|---|---|
8 | 650 | 648.7413 | -0.19% | - | - |
9 | 888 | 878.8429 | -1.03% | 1.366154 | 1.354689 |
10 | 1128 | 1167.422 | 3.49% | 1.27027 | 1.328363 |
11 | 1694 | 1525.264 | -9.96% | 1.501773 | 1.306523 |
12 | 2036 | 1964.633 | -3.51% | 1.201889 | 1.288061 |
13 | 2502 | 2499.426 | -0.10% | 1.22888 | 1.27221 |
14 | 3089 | 3145.342 | 1.82% | 1.234612 | 1.258426 |
15 | 3858 | 3920.067 | 1.61% | 1.248948 | 1.246309 |
16 | 4636 | 4843.463 | 4.48% | 1.201659 | 1.235556 |
17 | 5883 | 5937.786 | 0.93% | 1.268982 | 1.225938 |
18 | 7375 | 7227.909 | -1.99% | 1.253612 | 1.217274 |
19 | 9172 | 8741.575 | -4.69% | 1.243661 | 1.20942 |
20 | 10149 | 10509.65 | 3.55% | 1.10652 | 1.202261 |
21 | 12462 | 12566.43 | 0.84% | 1.227904 | 1.195704 |
22 | 15113 | 14949.91 | -1.08% | 1.212727 | 1.18967 |
23 | 17660 | 17702.14 | 0.24% | 1.16853 | 1.184097 |
24 | - | 20869.59 | - | - | 1.17893 |
25 | - | 24503.47 | - | - | 1.174123 |
26 | - | 28660.2 | - | - | 1.169638 |
27 | - | 33401.8 | - | - | 1.165442 |
28 | - | 38796.35 | - | - | 1.161505 |
29 | - | 44918.52 | - | - | 1.157803 |
30 | - | 51850.07 | - | - | 1.154314 |
31 | - | 59680.39 | - | - | 1.151019 |
32 | - | 68507.15 | - | - | 1.147901 |
33 | - | 78436.92 | - | - | 1.144945 |
34 | - | 89585.81 | - | - | 1.142138 |
35 | - | 102080.3 | - | - | 1.139469 |
36 | - | 116057.8 | - | - | 1.136927 |
37 | - | 131667.8 | - | - | 1.134502 |
38 | - | 149072.4 | - | - | 1.132186 |
39 | - | 168447.5 | - | - | 1.129971 |
40 | - | 189983.6 | - | - | 1.127851 |
Day # | Observed | Predicted | Error % | Day/Day O | Day/Day P |
---|---|---|---|---|---|
8 | 650 | 648.7413 | -0.19% | - | - |
9 | 888 | 878.8429 | -1.03% | 1.366154 | 1.354689 |
10 | 1128 | 1167.422 | 3.49% | 1.27027 | 1.328363 |
11 | 1694 | 1525.264 | -9.96% | 1.501773 | 1.306523 |
12 | 2036 | 1964.633 | -3.51% | 1.201889 | 1.288061 |
13 | 2502 | 2499.426 | -0.10% | 1.22888 | 1.27221 |
14 | 3089 | 3145.342 | 1.82% | 1.234612 | 1.258426 |
15 | 3858 | 3920.067 | 1.61% | 1.248948 | 1.246309 |
16 | 4636 | 4843.463 | 4.48% | 1.201659 | 1.235556 |
17 | 5883 | 5937.786 | 0.93% | 1.268982 | 1.225938 |
18 | 7375 | 7227.909 | -1.99% | 1.253612 | 1.217274 |
19 | 9172 | 8741.575 | -4.69% | 1.243661 | 1.20942 |
20 | 10149 | 10509.65 | 3.55% | 1.10652 | 1.202261 |
21 | 12462 | 12566.43 | 0.84% | 1.227904 | 1.195704 |
22 | 15113 | 14949.91 | -1.08% | 1.212727 | 1.18967 |
23 | 17660 | 17702.14 | 0.24% | 1.16853 | 1.184097 |
24 | - | 20869.59 | - | - | 1.17893 |
25 | - | 24503.47 | - | - | 1.174123 |
26 | - | 28224.73 | - | - | 1.151866 |
27 | - | 31390.92 | - | - | 1.112178 |
28 | - | 34800.89 | - | - | 1.108629 |
29 | - | 37472.46 | - | - | 1.076767 |
30 | - | 40467.4 | - | - | 1.079924 |
31 | - | 42952.6 | - | - | 1.061412 |
32 | - | 44982.47 | - | - | 1.047258 |
33 | - | 47993.6 | - | - | 1.06694 |
34 | - | 52150.91 | - | - | 1.086622 |
35 | - | 54307.97 | - | - | 1.041362 |
36 | - | 55948.02 | - | - | 1.030199 |
37 | - | 57620.74 | - | - | 1.029898 |
38 | - | 59162.79 | - | - | 1.026762 |
39 | - | 60591.3 | - | - | 1.024145 |
40 | - | 60910.65 | - | - | 1.005271 |
In the meantime, the country is facing substantial economic and social damage in several sectors. There has been a collapse in tourism, with estimated losses of around 7.4 billion euros, with a drop of 31.6 million visitors over the next 3 months [5]. Most shops have been forced to close, with a devastating impact on incomes. In the factories from north to south, there has been a wave of spontaneous strikes, with several labor unions subsequently taking the position that they consider necessary to stop all metalworkers, “regardless of the contract used, until Sunday, March 22nd. This, in order to “sanitize, secure and reorganize all workplaces ” [6]. While school closures help to mitigate the effects of the pandemic by reducing the rate of infection among children and adolescents, they also involve costs that should not be underestimated due to the forced absenteeism of some parents to take care of younger children, and in case of prolonged closure, this factor can also have a significant impact on GDP [7]. The Milan stock exchange on March 12th recorded a loss of 16.92%, the worst ever.
On March 17th, the “Cura Italia” decree was approved, the key points of which are: strengthening of the national health system, support for employment and workers, credit support for families and small/medium enterprises, suspension of taxes and other tax obligations.
The model clearly shows how useful containment measures, if implemented effectively, can be in the context of this specific pandemic in Italy. It is, therefore, necessary for the Italian health system to adopt such measures comprehensively and efficiently. Unfortunately, to date, there is insufficient data to state which trend, between the two hypotheses supplied by the model, the spread of the infection will follow. In both cases, given the considerable geographical concentration of the existing cases, the healthcare system is facing an unusual burden.
Based on the assumed numbers (especially in the worst-case scenario), it will be necessary to follow some healthcare policy and organizational measures. In particular, some are:
- Partial reconversion of low emergency wards, by equipping them with patient support structures wherever possible
- Development of advanced triage decision systems, specifically devoted to coronavirus
- Development or optimization of tools for the prioritization of patients
- Increased surface sanitation, potentially including innovative tools that appear to have proven effective against viruses and bacteria, such as ozone [8, 9]
- Creation of dynamic and structured networks on the territory.
ACKNOWLEDGEMENTS
The author would like to gratefully acknowledge dr. Francesco Tasso who helped him gather and select a huge amount of bibliographic resources in a few days and Dr. Elena Mondo, MD for helping collect and select some medical references.