All published articles of this journal are available on ScienceDirect.
Quantile Regression Analysis of Modifiable and Non-Modifiable Predictors of Stroke among Adults in South Africa
Abstract
Background:
Stroke is the second largest cause of mortality and long-term disability in South Africa (SA). Stroke is a multifactorial disease regulated by modifiable and non-modifiable predictors. Little is known about the stroke predictors in SA, particularly modifiable and non-modifiable. Identification of stroke predictors using appropriate statistical methods can help formulate appropriate health programs and policies aimed at reducing the stroke burden. This study aims to address important gaps in stroke literature i.e., identifying and quantifying stroke predictors through quantile regression analysis.
Methods:
A cross-sectional hospital-based study was used to identify and quantify stroke predictors in SA using 35730 individual patient data retrieved from selected private and public hospitals between January 2014 and December 2018. Ordinary logistic regression models often miss critical aspects of the relationship that may exist between stroke and its predictors. Quantile regression analysis was used to model the effects of each predictor on stroke distribution.
Results:
Of the 35730 cases of stroke, 22183 were diabetic. The dominant stroke predictors were diabetes, hypertension, heart problems, the female gender, higher age groups and black race. The age group 55-75 years, female gender and black race, had a bigger effect on stroke distribution at the lower upper quantiles. Diabetes, hypertension and cholesterol showed a significant impact on stroke distribution (p < 0.0001).
Conclusion:
Most strokes are attributable to modifiable factors. Study findings will be used to raise awareness of modifiable predictors to prevent strokes. Regular screening and treatment are recommended for high-risk individuals with identified predictors in SA.
1. INTRODUCTION
The World Health Organisation (WHO) defines stroke as a condition characterised by rapidly developing symptoms and signs of a local brain lesion, with symptoms lasting for more than 24 hours, or leading to death with no apparent cause other than that of vascular origin [1]. Stroke remains a leading cause of long-term disability and the second cause of death [2]. Stroke is becoming a major public health issue in Africa, yet little is known about modifiable and non-modifiable predictors of stroke [3]. Even though stroke can be prevented by treatment of modifiable risk factors, it remains one of the biggest threats to public health worldwide [4]. Prevention begins with the identification and raising awareness of stroke risk factors. To fill this gap, this study identified and quantified the effect of modifiable and non-modifiable predictors of stroke using a quantile regression approach in South Africa (SA).
In SA, stroke is the second leading cause of mortality after HIV/AIDS and is among the top ten leading causes of long-term disability [5]. Moreover, stroke is responsible for 25 000 deaths annually and 95 000 individuals live with disability in SA, yet only a few published studies report on the modifiable and non-modifiable predictors of stroke [5]. SA is undergoing an epidemiological transition driven by socio-demographic and lifestyle changes leading to an upswing of non-communicable diseases such as stroke [5, 6]. Knowledge on the relative contribution of modifiable and non-modifiable risk factors on stroke disease occurrence is needed for public health early awareness, prevention efforts and effective interventions.
Predictors of stroke can be classified as modifiable and non-modifiable, where modifiable factors are preventable e.g., hypertension, smoking, cholesterol, obesity and diabetes, whilst non-modifiable predictors are not preventable such as age, gender and race [7]. Most studies identified hypertension, cholesterol, heart problems, smoking, obesity and diabetes as major modifiable predictors and the male gender, higher ages and the black race as non-modifiable predictors [7]. However, in SA, most studies were focusing on modifiable predictors and identified hypertension, cholesterol and diabetes as critical modifiable predictors [5]. There is a need to know and quantify the prevalence and contribution of modifiable and non-modifiable stroke predictors in SA. This study aims to identify the prevalence of the most important modifiable and non-modifiable predictors using hospital-based data collected between January 2014 and December 2018 in SA. This study will allow the identification of vulnerable groups and other characteristics for possible early intervention.
Logistic regression is a common modelling technique for analysing disease risk factors in medical research. Logistic regression analysis focuses on the conditional mean only and the models can probably miss critical aspects of the relationship between risk factors and stroke. Most studies used the logistic regression technique to identify stroke predictors despite its limitation of focusing solely on the conditional mean [2], to the exclusion of relationships at the extremities. Although many researchers used ordinary logistic regression to model stroke predictors, very few studies used the quantile regression method to quantify the effect of predictors on stroke. This study identified and quantified modifiable and non-modifiable stroke risk factors by the use of ordinary logistic and classical quantile logistic regression techniques to understand the effect of each predictor on stroke distribution. Quantile Regression (QR) was used in this study because it is more appropriate in many situations than the mean regression, and it provides a detailed overview of the stroke distribution, including the relationships at the extremities. QR methods provide a more complete description of functional changes than focusing solely on the mean and it provides more comprehensive information on the relationship between the outcome variable and the covariates than the ordinary logistic regression.
2. MATERIALS AND METHODS
A cross-sectional study design was used to identify and quantify modifiable and non-modifiable predictors of stroke in SA for the data collected between January 2014 and December 2018. It is a descriptive epidemiological study in which the exposure and stroke disease status of the South African sub-population was determined at a given point in time. The study design chosen was aimed at attaining immediate knowledge and information about predictors of stroke. Confirmation of stroke was based on computed tomography or magnetic resonance imaging.
2.1. Study Variables
The study outcome variable was confirmed stroke coded, 1= yes and 0 = no. Whilst the explanatory variables were demographic characteristics of stroke patients, modifiable, and non-modifiable risk factors including age, gender, and race. The race variable in SA is categorised as whites, blacks, coloureds, Indians and Asians. This study combined Indians and Asians to be one category because of smaller numbers. Coloured is a person of mixed European (“white”) and African (“black”) or Asian ancestry (“brown”), as officially defined by the South African government from 1950 to 1991. Thus, Coloureds are a multiracial ethnic group native to SA who have ancestry from more than one of the various populations inhabiting the region / coloureds are a mixed race group in SA. They are dominant in the Western Cape province of South Africa.
2.1.1. Independent Variables
Diabetes was defined as a fasting glucose concentration of greater than 7.0 mmol/L, cholesterol was defined as fasting cholesterol concentration of at least 5.2 mmol/L, high-density lipoproteins cholesterol at least 1.03 mmol/L and low-density lipoproteins cholesterol of at least 3.4 mmol/L. Whilst hypertension was defined with cut off of 140/90 mmHg for up to 72 hours and heart problems were defined as current atrial fibrillation, heart failure, ischemic heart disease, and valvular heart diseases [2, 8].
The modifiable risk factors of stroke were hypertension, cholesterol, heart problems, and diabetes coded as 0 = no, if the measurement is below the defined value of interest and 1 = yes, if the measurements exceeded the study definition.
2.2. Data
The study sites consist of the nine provinces of SA with an estimate of a mid-year population of 57.73 million [9]. There are approximately 407 public and 203 private hospitals in SA [10]. This study randomly selected 55% of the 203 private hospitals in all provinces and 45% of the 407 public hospitals were randomly selected across nine provinces of SA. A stratified probability sampling technique was used to calculate the proportions accordingly. The strata being public and private hospitals. Thus, study data were retrieved from 183 public and 112 private hospitals, making a total of 295 hospitals. Although most South Africans use public hospitals, many of these institutions did not capture good quality pertinent variables while private hospitals were doing so. The proportions and final variables used were based on the availability of study variables in public and private hospital databases and the total number of private and public hospitals in SA. Therefore, 55% of the data were retrieved from private hospitals and 45% from public hospitals.
A validated data retrieval sheet was used to retrieve study data. Patients’ medical records were reviewed to elicit all predictors of stroke. The data retrieval sheet was formulated with all the study variables, which include; confirmation of stroke, and stroke predictors that are non-modifiable and modifiable. The variable type of hospital, that is, private or public hospital, was anonymous for ethical reasons which means there was no variable specifying the type of hospital admitted as agreed upon in advance. The study hospitals were sampled from the nine provinces of SA namely Gauteng, KwaZulu-Natal, Western Cape, Eastern Cape, North West, Free State, Limpopo, Mpumalanga, and Northern Cape. The case managers for the sampled hospitals assisted with data retrieval. The total number of stroke patients was 35730. There was no missing information in the selected final variables for every patient, which implies that there were no patients with partial or no information.
2.3. Statistical Analyses
Descriptive analyses were conducted to describe stroke patients’ characteristics using frequencies and their associated percentages for categorical variables. Since there was no variable for the type of hospital (i.e., public/private), data analysis was not done for public or private hospitals separately. All analyses were done in R statistical software version 4.0.2. The R add-on package quantreg was used for fitting the multivariate QR model. QR analysis was employed to assess the effect of modifiable and non-modifiable predictors on stroke distribution. Modelling of stroke predictors was done to develop a predictive and a descriptive model. QR analysis was employed because it gives much more information about the underlying associations, is not robust to outliers, and provides flexibility in analysing the predictors of stroke corresponding to quantiles of interest either in the lower tail, the central location or the upper tail of the distribution rather than investigating only the predictors of the mean distribution.
The study logistic regression model for modifiable and non-modifiable stroke predictors is given as:
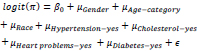
where π=P(y=1) is the probability of developing stroke, μGender is the gender effect on stroke, μAge-category is the age category effect on stroke, μRace is the race effect on stroke, μHypertesion - yes is the hypertension effect on stroke, μ Cholester o l-yes is the cholesterol effect on stroke, μDiabetes -yes is the diabetes effect on stroke, and ϵ is the error term.
The study logistic regression model for non-modifiable predictors can be re-expressed in terms of the βj’s.
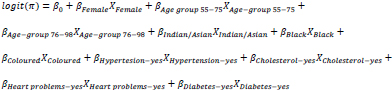
The reference is a relatively young (18-54 years old) white male without any of the problems hypertension, cholesterol, heart problems and diabetes.
Let Y be the outcome of interest (i.e., confirmed stroke in this case) and X a vector of observed covariates. We can model quantile of Y conditional on X= x using a quantile logistic regression model given as:

where π = P(y = 1) is the probability of developing stroke, Yi is the ith confirmed stroke individual, β 0(τ) is the intercept for the given quantile, β1...βp are the other p unknown parameters of each quantile, Xi1,... Xip are the known p independent covariates for the patient i, and it is the dummy variables associated with (gender (2 categories), age group (3 categories) and race (4 categories), (hypertension, cholesterol, heart problems, diabetes, with two levels each respectively)), and ϵ (τ) is the error term associated with patient i, and is the 0.1, 0.25, 0.50, 0.75 and 0.95 quantiles. The formulation in (3) permits the modelling of two or more quantiles of stroke modifiable and non-modifiable predictors simultaneously while adjusting for the observed covariates.
2.4. Ethical Considerations
Permission to conduct this research was obtained from the Provincial Health Departments and from individual hospitals. The research was granted permission by the committee of research on human subjects of the University of South Africa as well as the study hospitals. The ethical clearance reference number is 2017/SSR-ERC/001.
3. RESULTS
The results of the analysis are summarised in this section. The demographic and baseline characteristics of the stroke patients are given first.
Table 1 depicts the demographic and some selected background characteristics of stroke patients in SA. Most of the stroke patients were relatively young (18-54 years), thus an indication of more young strokes 19474/ 100 000. The dominant racial groups who suffered a stroke were whites (34.6%) and blacks (29.6%) and the last group was coloureds (14.6%). They are marginally more females (50.8%) than males. The major modifiable stroke predictors were diabetes (62.1%), hypertension (55.3%) and heart problems (54.4%). Of the 35 730 patients, 77.1% had an ischemic stroke.
Table 2 shows the magnitude of the association between stroke and its predictors. As mentioned before, the reference is a relatively young (18-54 years old) white male without any of the problems hypertension, cholesterol, heart problems and diabetes. With this basis, model parameters are positive. Further, the odds ratio for females compared to males is 1.16. This entails that females have a 16% higher risk of developing stroke than males in South Africa. Further, the findings in Table 2 depict that the odds ratio is 1.16 for those aged 55-75 years when compared to the reference (18-54 years age group). This implies that, for the age group 55-75 years, the risk of developing stroke is 16% times higher than the reference age group. The odds ratio of patients aged between 76 and 98 years developing stroke when compared to the basis is 62% higher. The odds ratio of black people developing stroke when compared to whites is almost 7 fold higher. This means blacks are much more at risk of developing a stroke than whites in South Africa. Additionally, the odds ratio for the group Indians/Asians compared to whites is 1.16. This group has a 16% higher risk of developing stroke than whites in South Africa. Lastly, the log odds of coloureds, when compared to whites, is 22.089, which is very high, but the coefficient is not significant. All other coefficients are significant. The risk for coloureds to develop stroke is the same as the whites, but there seems to be a lot of variation within the coloured grouped as evidenced by the very high variance for β with a variance of 558.59.
The parameters in this simple model, of having hypertension, cholesterol, heart problems and diabetes are positive and significant, thus confirming their greater impact on the risk of stroke distribution. The log odds of people suffering a stroke due to hypertension is 0.32 when compared to those without hypertension. The coefficient is positive, which means hypertensive individuals are more at risk of developing stroke than people without hypertension in South Africa. The odds ratio is 1.38 when comparing people with hypertension to those without. The odds for hypertensive people developing stroke are approximately 38% higher than the odds for those without hypertension.
Moreover, the odds of patients with cholesterol developing stroke when compared to the basis is 87% higher. This means individuals with cholesterol are much more at risk of suffering stroke than those without cholesterol in this South African population. Further, the odds ratio for the people with diabetes compared to those without diabetes is 5.03. These patients have a 403% higher risk of developing stroke than those who are not diabetic in South Africa. Study findings also indicate that the log odds of people with heart problems developing a stroke is 0.16, implying that individuals with heart problems are more at risk of developing stroke than those without heart problems in SA. All coefficients are significant, meaning the effect of these modifiable factors on stroke was significant.
Variable | n(%) |
---|---|
Age group 18-54 years 55-75 years 76-98 years |
19474 (54.5) 10446 (29.2) 5810 (16.3) |
Gender Male Female |
17565 (49.2) 18145 (50.8) |
Race Black White Indian/Asian Coloured |
10560 (29.6) 12243 (34.3) 7724 (21.6) 5203 (14.6) |
Hypertension Yes No |
19756 (55.3) 15974 (44.7) |
Heart problems Yes No |
19453 (54.4) 16277 (45.6) |
Diabetes Yes No |
22183 (62.1) 13547 (37.9) |
Cholesterol Yes No |
16923 (47.4) 18807 (52.6) |
Type of stroke Ischemic Hemorrhagic |
27550 (77.1) 8180 (22.9) |
Variable | Coefficient | 95% Confidence Interval(CI) | Odds Ratio | P-value |
---|---|---|---|---|
Intercept | -2.248 | -3.148, -0.849 | 0.11 | 0.000 |
Age group 55-75years | 0.145 | 0.100, 0.246 | 1.16 | 0.000 |
Age group 76-98years | 0.479 | 0.399, 0.540 | 1.62 | 0.000 |
Female-gender | 0.147 | 0.140, 0.150 | 1.16 | 0.000 |
Indian/Asian-race | 0.150 | 0.140, 0.200 | 1.16 | 0.000 |
Black-race | 1.850 | 0.850, 2.189 | 6.36 | 0.000 |
Coloured-race | 22.089 | -22.000, 23.088 | 3918605310 | 0.967 |
Hypertension-yes | 0.321 | 0.300, 0.350 | 1.38 | 0.000 |
Heart problems-yes | 0.162 | 0.1567, 0.165 | 1.18 | 0.000 |
Cholesterol-yes | 0.628 | 0.455, 0.630 | 1.87 | 0.000 |
Diabetes-yes | 1.617 | 1.589, 1.620 | 5.04 | 0.000 |
Variable | τ=0.10 | - | τ=0.25 | - | τ=0.50 | - | τ=0.75 | - | τ=0.95 | - |
---|---|---|---|---|---|---|---|---|---|---|
- | βj |
OR: exp(βj) |
βj |
OR: exp(βj) |
βj |
OR: exp(βj) |
βj |
OR: exp(βj) |
βj |
OR: exp(βj) |
Intercept | -1.707*** | -1.688*** | -1.091*** | -1.644*** | -1.832*** | |||||
Age group-55-75years | 1.911*** | 6.76 | 2.844*** | 17.89 | 2.252*** | 9.51 | 1.991*** | 7.32 | 2.565 | 13.00 |
Age group-76-98 years | 2.132 | 8.43 | 3.530 | 34.12 | 2.279 | 9.77 | 2.471 | 11.83 | 1.184 | 3.27 |
Female gender | 1.437 | 4.21 | 2.278 | 9.76 | 1.556 | 4.74 | 1.665 | 5.29 | 2.144 | 8.53 |
Indian/Asian-race | 1.881 | 6.56 | 2.497 | 12.15 | 2.229 | 9.29 | 2.449 | 11.58 | 2.154 | 8.62 |
Black-race | 2.114 | 8.28 | 1.079 | 2.94 | 2.604 | 7.88 | 2.156 | 8.64 | 2.184 | 8.88 |
Coloured-race | 2.343 | 10.41 | 1.787 | 5.97 | 1.796 | 6.03 | 2.714 | 15.09 | 2.144 | 8.53 |
Hypertension-yes | 1.823 | 6.19 | 3.015 | 20.39 | 1.509 | 4.52 | 2.111 | 8.26 | 1.154 | 3.17 |
Heart-problems-yes | 1.758 | 5.80 | 2.525 | 12.49 | 0.777 | 2.17 | 1.768 | 5.86 | 2.777 | 16.07 |
Diabetes-yes | 2.897 | 18.12 | 2.590 | 13.32 | 1.873 | 6.51 | 1.813 | 6.13 | 2.495 | 12.12 |
Cholesterol-yes | 1.565 | 4.78 | 1.909 | 6.75 | 0.759 | 2.14 | 2.037 | 7.67 | 2.278 | 9.76 |
It is evident from Table 3 that female gender, black and Indian/Asian, hypertension, cholesterol, heart problems and diabetes are significantly associated with stroke across quantiles. In multivariate quantile regression analysis, the effect of the age group 55-75 years on stroke is significantly stronger at the 95th quantile than at the 10th,25th, 50th and 75th quantiles. Therefore, the magnitude of association for the age group 55-75 years increases from low to high quantiles. Also, the risk of suffering stroke for individuals aged 76-98 years is 9.77 times higher than those aged 18-54 years at the 50th quantile. Study results show that the black race effect on stroke is much larger at the upper end than at the lower end. Overall, the estimated conditional quantile functions for all non-modifiable predictors significantly increase from low to upper quantiles except for the coloured race. These positive significant coefficients entail that the impact of the female gender, black race, Indian/Asian race and higher age groups on stroke is bigger at the central location and upper quantiles compared to lower quantiles (Table 3).
With regards to modifiable predictors of stroke, the effect is positive and significant across quantiles. The magnitude of association for modifiable factors with stroke fluctuates across the quantiles. The risk of developing a stroke due to hypertension is 8.26 times higher at the 95th than those without hypertension. The findings also indicated that being diabetic is positively associated with stroke across the quantiles. These positive significant coefficients imply that the risk of developing a stroke is likely to increase in people with elevated diabetes levels. The estimated conditional quantile functions for heart problems fluctuate across quantiles with a bigger effect at the 95th quantile. Lastly, the effect of cholesterol on stroke is greater at the 95th quantile and smaller at the 10th quantile. Thus, the magnitude of association increases from the low end to the upper end of the stroke distribution. Largely, the effect of hypertension, cholesterol and diabetes increase from the lower to the upper quantiles of the stroke distribution. Thus, people with elevated hypertension, cholesterol and diabetes are likely to have a stroke relative to those without these risk factors. All coefficients are significant, meaning the effect of these modifiable factors on stroke is significant (Table 3).
4. DISCUSSION
This paper identified and quantified the modifiable and non-modifiable predictors of stroke in SA using quantile regression to elucidate the differential effects of each putative predictor on stroke. As anticipated, risk factors such as female gender, higher age groups, black and Indian/Asian races, hypertension, cholesterol, heart problems and diabetes differently affected stroke patients at each quantile. The findings showed that the female gender had a higher effect on stroke across all the quantiles. The risk of developing stroke was significantly higher in women than in men because of their longer life span and much higher incidence at older ages. Reeves et al. [11] also found that stroke had a greater effect on women than men because of their longer life expectancy. A study conducted in the United States of America established that there were more strokes in women than in men due to sex hormones and longer life expectancy [12]. Further, an American study indicated that as women age, they suffer a stroke due to loss of estrogen with menopause [13]. Thus, the risk of stroke in elderly women surpasses that of men. In a study by Horsten et al. [14], the risk of stroke was found to be high in very old women with high blood pressure. Studies in various parts of the world have found differences in gender stroke incidence [15]. Since the risky gender group has been identified in SA, this study recommends campaign services on raising awareness of the dangers of stroke risk, targeting the female gender.
Despite women being at an increased risk of suffering stroke, some studies identified male gender being at higher risk of suffering stroke than women possibly due to unhealthy lifestyles habits in men such as smoking, alcohol consumption and physical inactivity leading to obesity [16]. Previous studies have found differences between sexes in stroke incidences and revealed that the most common biological explanation for gender differences in stroke was the presence of sex hormones [16]. Future research is needed to determine whether the pathology of a stroke differs between men and women. Stroke is known as a disease of ageing and the incidence of stroke doubles after the age of 55 years [17]. The incidence of stroke increases with age possibly due to the physiological, pathological and social changes associated with ageing [17]. Consistent with other study findings, this study also reported an increased incidence of stroke in higher age groups 55-75 and 76-98 years. The impact of age on stroke found by Gan et al. [18] shows that people above 55 years were 1.87 times at higher risk of suffering stroke than young people. However, Howard et al. [19] found younger female strokes in the black race and elevated hypertension. The other reasons for younger age female strokes could be related to pregnancy, post-partum state and hormonal factors such as hormonal contraceptives [17]. The higher risk of stroke in black people could be due to the high prevalence of hypertension and becomes more important with increasing age [20]. The determinant factors for hypertension in blacks could be genetic, high salt intake, poverty and availability of cheap and unhealthy diets in SA [21]. An American study on stroke risk factors also found higher stroke incidence in black adults due to a higher prevalence of hypertension, diabetes and obesity than in white adults [17]. Goldstein et al. [22] had a similar conclusion that blacks have high stroke incidence than whites. Middle-aged blacks also showed a substantially higher risk of stroke than whites of similar ages in an American study by Choudhury et al. [16].
Indians/Asians have a higher risk of developing a stroke than whites in South Africa, possibly due to the prevalence of cholesterol problems in the Indians population group. Similar findings were reported by Boehme et al. and Goldstein et al. that Chinese, Japanese and Indian people had higher stroke incidence compared to white people [17, 22]. An American study found more incidence of stroke in black females due to low socio-economic status than whites [23]. The risk for coloureds developing a stroke is the same as the whites, but there seems to be a lot of variation within the coloured group. In SA, the prevalence of smoking is greater in female coloureds than males and is one of the possible reasons for high stroke variance in the coloured population compared to whites [23]. Deliberate efforts may reduce the stroke burden in SA by targeting vulnerable racial groups, age groups and gender. Reducing the burden of stroke in the SA population requires the identification of non-modifiable predictors of stroke and demonstration of the efficacy of the risk reduction. Stroke is a multi-factorial disorder. Several predictors are associated with an increased risk of stroke [7, 8]. These predictors are classified as modifiable and non-modifiable.
Another important study finding was that modifiable predictors such as hypertension, cholesterol, heart problems and diabetes were found to be significantly associated with stroke. The results of this study are consistent with a Jordanian study that identified hypertension, diabetes and heart problems as the most common predictors of ischemic stroke [24]. Boehme et al. [17] also found that hypertension, diabetes and cholesterol as the most important modifiable predictors for stroke due to obesity and physical inactivity, leading to a higher incidence of stroke. Hypertension usually increases with ageing [17].
In SA, hypertension is the most prevalent modifiable risk factor of stroke. The prevalence of hypertension increases with age in black South Africans mainly due to: high urbanisation with the adoption of Westernised food and lifestyles leading to bad dietary habits, physical inactivity and obesity [21]. Other possible reasons for the high prevalence of hypertension leading to more strokes could be excessive salt intake, genetic factors and alcohol consumptions in black South Africans [20]. Hornsten et al. [14] also found that high blood pressure was the major risk factor for stroke in their cohort study due to social changes associated with ageing. Similar findings on hypertension being the most prevalent modifiable risk factor for stroke was reported in an American study [4]. It is therefore important for early detection and treatment of hypertension in SA to reduce the burden of stroke. Future clinical trials focusing on treating blood pressure at earlier stages are urgently needed in SA.
There were some limitations to this study. Predictors such as obesity, HIV/AIDS, smoking and alcohol consumption were not captured in the patient’s records. Family history of stroke and genetics were not available in the data, yet they are important predictors of stroke. Nevertheless, the strengths of this hospital-based study are that recent data set has been used without missing information. To date, this is the only comprehensive cross-sectional study design that was used to identify and quantify modifiable and non-modifiable predictors of stroke in SA using the quantile regression modelling technique. According to the authors’ knowledge, this is the only study that included all stroke predictors i.e., modifiable and non-modifiable predictors and examining all predictors for a more comprehensive evaluation of stroke predictors in SA.
CONCLUSION
In summary, the female gender, age groups 55-75 and 76-98 years, black and Indian/Asian racial groups, hypertension, cholesterol, heart problems and diabetes had a greater impact and significant effect on stroke distribution. The study findings showed that strokes were attributable to established modifiable predictors and could be prevented by an early intervention system such as regular screening and treatment of hypertension, cholesterol, heart problems and diabetes. The significant modifiable predictors in SA are diabetes, hypertension, heart problems and cholesterol. The study recommends regular screening and testing and treatment of hypertension, cholesterol, heart problems and diabetes in SA in the black population, in particular, to detect the risk of stroke early enough.
LIST OF ABBREVIATIONS
QR | = Quantile Regression |
CI | = Confidence Interval |
OR | = Odds Ratio |
SA | = South Africa |
AUTHOR'S CONTRIBUTIONS
LM contributed towards the conceptualisation of the study, study design, literature search, collected data and prepared it for analysis, analysed data and interpreted the results and manuscript write-up. DC critically reviewed and corrected misconceptions in the final version of the revised manuscript and approved the final manuscript. All the authors have read and approved the final version of the manuscript and agreed to be accountable for all aspects of the work.
ETHICS APPROVAL AND CONSENT TO PARTICIPATE
Ethical approval for this study was granted by the committee of research on human subjects of the University of South Africa and the reference number is 2017/SSR-ERC/001. Administrative permission was obtained directly from the hospital to access the data. Written informed consent forms were obtained from the hospital managers before data retrieval from patients’ medical records.
HUMAN AND ANIMAL RIGHTS
No animals were used in this research. All human research procedures were followed in accordance with the ethical standards of the committee responsible for human experimentation (institutional and national), and with the Helsinki Declaration of 1975, as revised in 2013. (i.e., patients’ rights were adhered to by not using patient names, IDs in reporting study results and also hospital names were not used in the data analysis as agreed in advance during the ethical clearance application process).
CONSENT FOR PUBLICATION
The objectives of the study were explained to the selected study hospital managers. They were ensured about the confidentiality of information, and were asked to complete the informed consent.
AVAILABILITY OF DATA AND MATERIALS
The datasets used or analysed during the current study are not available from the corresponding author to share with the public because the hospital managers do not permit it for ethical reasons as agreed in advance.
FUNDING
This research study received funding from the National Research Foundation (NRF) South Africa. The grant reference number is: SFH16069956
CONFLICT OF INTEREST
The authors declare no conflict of interest, financial or otherwise.
ACKNOWLEDGEMENTS
We would like to thank the authorities of respective hospitals who supplied their data and for their tremendous support during this study. The authors thank the National Research Foundation (NRF) for funding this project.