All published articles of this journal are available on ScienceDirect.
Machine Learning Guided Lyric-analysis Peer Support Intervention for Psychological Distress in African Population: A BOM Conceptualized Framework
Abstract
Background
Sub-Saharan Africa faces a significant burden of mental health challenges, including depression and anxiety, particularly among vulnerable populations such as women and individuals living with HIV. This study proposes a machine learning-guided Lyric Analysis Peer Support Intervention (LAPSI) to predict psychological distress and inform interventions. The study utilizes machine learning to generate risk profiles, presenting an innovative application in identifying psychological distress risk factors for developing targeted interventions.
Objective
The objective of this study is to utilize machine learning models to generate risk profiles that predict psychological distress among HIV-positive and HIV-negative populations and to use these profiles to guide thematic development for LAPSI, ensuring confidentiality and informed consent.
Methods
The study aims to leverage machine learning algorithms such as Logistic Regression and Random Forest to generate risk profiles and to analyze demographic, socio-economic, and psychological factors, including HIV status, age, education, and employment. Introducing a novel application of machine learning in the identification of psychological distress risk factors for intervention development while adhering to ethical standards for handling sensitive data like HIV status. Prior to data collection, ethical approval will be obtained, ensuring participant confidentiality and informed consent. Themes generated from the risk profiles will inform song selection and peer support intervention for LAPSI.
Results
The machine learning models are expected to highlight education, employment status, and marital status as critical predictors of psychological distress. Geographical and demographic variables, such as district and age, are hypothesized to play a significant role in predicting distress among both HIV-positive and HIV-negative cohorts.
Conclusion
This study posits that machine learning models will provide actionable insights into predicting psychological distress, enabling targeted interventions through LAPSI. This short communication argues for integrating LAPSI into health policies and calls upon health policy actors to recognize its potential in addressing the mental health crisis in Sub-Saharan Africa, advocating for partnerships with local communities, healthcare providers, and mental health advocates to tailor and implement this intervention effectively.
1. INTRODUCTION
Lyric analysis peer support intervention (LAPSI) is an innovative machine learning-guided approach proposed in this communication to address mental health challenges directly in Sub-Saharan Africa (SSA). In SSA, mental health challenges are a significant public health concern, with a high prevalence of conditions such as depression and anxiety [1, 2]. Lack of access to quality mental health care worsens these conditions, particularly for vulnerable populations such as women. This population constantly faces unique barriers to accessing mental health care as a result of sociocultural norms that disfavor women, economic disempowerment, and Gender-Based Violence (GBV) [3, 4]. In this context, innovative ways that capitalize on existing community resources are critical and necessary to address this urgent public health concern [1]. One such approach is the Machine Learning Guided Lyric Analysis Peer Support Intervention (LAPSI). Moreover, by leveraging machine learning models to predict psychological distress and inform thematic development for lyric analysis, LAPSI offers a culturally sensitive and data-driven solution for mental health care in the region.
2. MATERIALS AND METHODS
2.1. Lyric Analysis in Mental Health Care
Lyric analysis is an approach in mental health care that is a promising approach because of its ability to facilitate emotional expression and communication. Analyzing song lyrics can help individuals connect with their inner feelings, process difficult emotions, and develop self-awareness [5]. Successful lyric analysis interventions have been utilized in various mental health settings, showing their prospective therapeutic ability [6]. Lyric analysis has been utilized to facilitate emotional expression and reflection in therapeutic settings. For example, one study provided a framework for the group process of lyric analysis intervention in a mental health setting [7]. Though there are criticisms for approaches like lyric analysis, such as the subjective nature of music interpretation, the challenge of quantifying therapeutic outcomes, and the difficulty of applying a standardized method to lyric analysis across diverse cultural contexts, Silverman (2009) found that lyric analysis could help individuals articulate feelings and experiences, potentially offering therapeutic benefits. The lyric analysis is traditionally rooted in music therapy, which catalyzes personal insight and emotional release. Evidence has exemplified how analyzing and discussing song lyrics can help individuals process complex emotions and experiences [8, 9]. A study [10] demonstrates how lyrics can mirror personal narratives of struggle and survival, thereby assisting in the therapeutic process. While these authors show the potential of lyric analysis in reflecting personal narratives, the method may not resonate with everyone. It could be less effective for those who do not have a natural affinity for music or for whom the music selected does not align with their personal experiences. Nevertheless, when appropriately applied, lyric analysis remains a powerful tool that can induce introspection and promote healing.
2.2. Peer Support in Mental Health Care
On the other hand, peer support can play a vital role in enhancing mental health outcomes and is considered a valuable addition to traditional approaches. It helps create a supportive environment and diminishes the stigma associated with mental health, empowering individuals to handle their challenges better. Mental health care systems have effectively incorporated peer support programs, providing a cost-efficient and culturally appropriate remedy. Peer support involves individuals with lived experiences providing support for each other, which can foster a sense of empathy and shared understanding [11]. It is built on the premise of mutual assistance among individuals with shared experiences and has been validated in the literature for its effectiveness in improving mental health outcomes. Davidson et al. emphasized the function of peer support in fostering empowerment, reducing symptoms, and enhancing recovery for individuals with mental health conditions. Furthermore, a study [12] underscores that peer support can instill hope and promote a shared understanding, which can be particularly valuable in managing HIV-related stigma and adverse mental health outcomes. However, the usefulness and success of peer support can vary significantly with the cultural context and individual personalities involved, influencing whether such intervention leads to positive health outcomes or unintentionally reinforces negative stigma. Despite these critical considerations, the core ethos of peer support-inherent empathy, empowerment, and shared experiences-remains a potential tool for mental health care. It plays a crucial role in supplementing traditional interventions with a compassionate, culturally sensitive approach that can resonate deeply with individuals, particularly in the context of adverse mental health outcomes. The technical contribution of this study lies in the novel application of these machine learning models in a mental health intervention framework. The prediction models will incorporate various factors, including demographic aspects (such as education and marital status), socioeconomic elements (like employment status and district name), and biological factors (for instance, HIV status), among others. While machine learning models have been used in disease prediction, this study introduces their application in predicting psychological distress and integrating these insights into the Lyric Analysis Peer Support Intervention (LAPSI). By applying SHAP (Shapley Additive Explanations), the study enhances the interpretability of machine learning predictions, ensuring transparency in understanding how different socio-economic and psychological variables impact distress [13]. A key consideration in applying ML to public health and mental health outcomes is the need for models that can accommodate the unique challenges presented by these data sets, including class imbalance, where instances of one outcome class significantly outnumber the other [13]. The ethical approval has been granted by the Sefako Makgatho University Research Ethics Committee (SMUREC): SMUREC Project ID:2139. Informed consent will be obtained from all participants before any data collection. Special care will be taken to ensure the confidentiality of personal and sensitive data, including participants' HIV status. Data will be anonymized and stored securely, ensuring that only authorized personnel have access.
2.3. Experimental: Data Mining and Development of MLG-LAPSI
The study will gather data from both HIV-positive and HIV-negative individuals, focusing on various aspects such as demographic information (like age and race), socio-economic background (including education and employment status), psychological factors (such as experience with intimate partner violence), biological details (HIV status), and environmental considerations (like the impact of HIV-stigma). The risk profiles generated by the machine learning models will then be used to inform the development of the Lyric Analysis Peer Support Intervention. Education, marital status, employment status, and district are expected to be the main variables influencing psychological distress. Based on these risk profiles, appropriate themes will be developed for peer support, guiding the selection of songs that resonate with participants. Additionally, by combining the emotional exploration and self-reflection enabled by lyric analysis with the encouraging community and diminished stigma of peer support, LAPSI establishes a comprehensive setting for individuals to manage their mental health progress. Exploring the potential of integrating lyric analysis within the framework of peer support can be adapted to peer support group settings where lyric analysis is used to promote self-expression and reflection on personal experiences related to mental health.
One study determined the effectiveness of using music and lyrics analysis with adolescents who have experienced complex developmental, and it was found that the method was an effective intervention used with the adolescents to express themselves and form self-identities while also talking about positive and negative relationships amongst each other in a group treatment setting [14]. Another study also explored the application of lyric analysis beyond its impact on mental health, finding its usability in fostering self-esteem, enhancement of coping mechanisms, and empowerment in songwriting and analysis of lyrics.
2.4. Clinical and Policy Implication of the Study
Findings from the music therapy methods showed a notable improvement in scores from the Rosenberg Self-Esteem Scale (RSES) before and after engaging in sessions with musical and non-musical interventions, with an alpha level of 0.20 (p < 0.20) [15]. The variations in each treatment were not sufficiently significant to be statistically meaningful, indicating that the effectiveness of each treatment was similar. Nonetheless, music therapy sessions consistently garnered higher attendance rates, suggesting greater interest among participants in music-based interventions than talk-based activities. Qualitative feedback was overwhelmingly positive, with participants expressing appreciation for the opportunity to express themselves and alleviate stress [15]. Also, the limitation of the study was the small sample size they had, which means the impact could be analyzed with a bigger sample size. The LAPSI seeks to synthesize these two evidence-based concepts, lyric analysis and peer support, creating an intervention that captures the therapeutic benefits of shared musical experiences within a supportive group context. This novel intervention could foster emotional expression, shared understanding, and social support, essential for mental health resilience and empowerment.
3. DISCUSSION
The proposed BOM conceptual framework (See Fig. 1) aims to identify key features or variables for developing LAPSI. The technical contribution of this study is in applying machine learning models to predict psychological distress and using these insights to inform a mental health intervention framework. In addition, utilizing embedded methods such as Lasso Regression, Random Forest, Decision Trees, and Gradient Boosting can help rank the importance of features based on their contribution to reducing error during the training of the model. Additionally, SHapley Additive Explanations (SHAP) for novel feature identification significantly enhance transparency in machine learning model predictions. Moreover, by assigning importance values to each feature based on their contribution, SHAP not only improves model transparency but also pinpoints critical predictors of distress, offering a unique contribution to the field [16]. While machine learning is widely used in disease diagnosis, its application in predicting psychological distress and shaping interventions in this context is a key advancement.
Additionally, by generating risk profiles based on socio-economic and demographic predictors, this study provides a data-driven foundation for LAPSI. The use of SHAP enhances the transparency of the models, allowing for a better understanding of the predictors influencing distress. These insights will guide the selection of songs and thematic interventions, offering participants a culturally relevant and emotionally engaging method of addressing psychological distress.
Additionally, the framework illustrates the integration of machine learning predictions, qualitative insights, and quantitative assessments to develop and refine LAPSI for Psychological Distress. The initial song selections and themes will be derived from the features and risk profiles the machine learning model identified. Additionally, this approach ensures that the intervention starts with a solid, data-driven foundation. Additionally, conducting interviews with a small sample of participants to refine and validate the initial theme and song selection will provide insights and ensure the intervention maintains a cultural context without requiring extensive resources. After the baseline data has been established, feedback on the impact of LAPSI, perceived motivators, and barriers will be conducted.
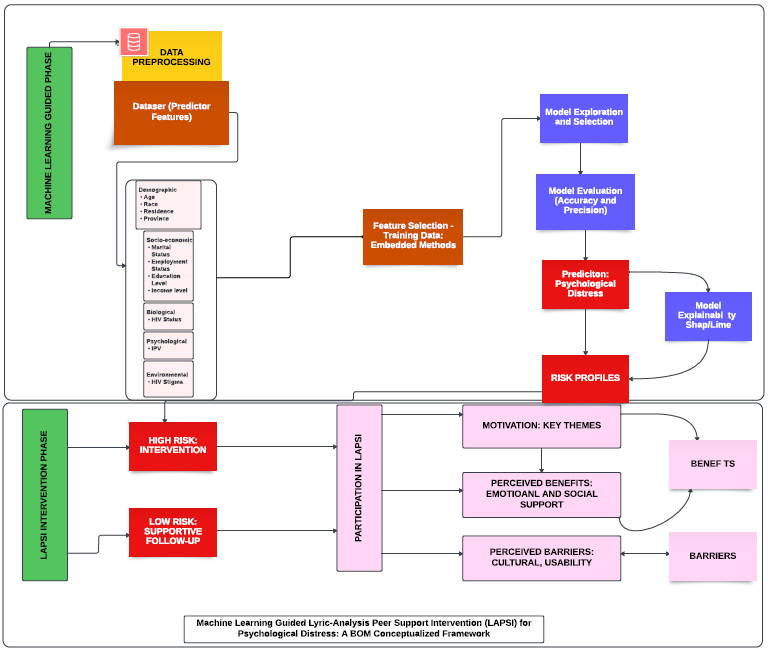
A BOM conceptualized LAPSI framework: Banda-oladimeji-mokgatle MLG-LAPSI framework (2024).
Successful implementation of the machine learning-guided LAPSI approach in SSA would necessitate thoughtful attention to the unique cultural and socioeconomic context. Forming partnerships with local community leaders, healthcare providers, and mental health advocates is essential to customizing the intervention to the target population's specific needs. Moreover, to ensure the successful adoption and sustainability of LAPSI, it is critical to provide training and empowerment to peer support workers and integrate LAPSI into the existing mental health care systems [17-21]. As health policy actors in SSA explore ways to address the growing challenges of adverse mental health, the LAPSI approach presents an innovative and exciting prospect to take of community human resources and empower individuals to manage their depression and anxiety.
CONCLUSION
In conclusion, this study posits that machine learning models will generate actionable insights into psychological distress, which can be used to inform thematic development for LAPSI. The machine learning-guided LAPSI presents a groundbreaking approach to mental health care in SSA. Additionally, by harnessing the therapeutic power of lyric analysis and the supportive nature of peer groups, LAPSI offers a culturally sensitive and holistic method to address critical mental health challenges faced by the SSA population. This intervention facilitates emotional expression and self-awareness through engagement with music, promotes solidarity, and reduces the stigma associated with mental health issues. Health policy actors must recognize the potential of LAPSI in bridging the gap in mental health services, particularly for vulnerable groups such as women who face numerous barriers in accessing care. There is a crucial need for collaboration among local communities, healthcare providers, and mental health advocates to tailor and implement LAPSI effectively, ensuring it is accessible to those who need it the most. Integrating LAPSI into health policies can improve mental health outcomes and overall well-being in SSA.
AUTHORS' CONTRIBUTION
It is hereby acknowledged that all authors have accepted responsibility for the manuscript's content and consented to its submission. They have meticulously reviewed all results and unanimously approved the final version of the manuscript.
LIST OF ABBREVIATIONS
MSCs | = Mesenchymal Stem Cells |
GBV | = Gender-Based Violence |
RSES | = Rosenberg SelfEsteem Scale |
SHAP | = SHapley Additive Explanations |
ETHICS APPROVAL AND CONSENT TO PARTICIPATE
The ethical approval has been granted by the Sefako Makgatho University, South Africa Research Ethics Committee (SMUREC): SMUREC Project ID:2139.
HUMAN AND ANIMAL RIGHTS
No animals were used that are the basis of this study. All the human procedure ware performed in accordance with the Helsinki declaration.
CONSENT FOR PUBLICATION
Informed consent will be obtained from all participants before any data collection.
FUNDING
Walter Sisulu Research Directorate is the one that will be paying the APC. There is no funding number.
ACKNOWLEDGEMENTS
Declared none.