All published articles of this journal are available on ScienceDirect.
Spatial Distribution and Determinants of Home Delivery of Women in Rural Ethiopia
Abstract
Introduction
Maternal mortality remains a global public health issue, with 303,000 women dying each year due to childbirth-related complications. In Ethiopia, it is common practice for women to give birth without a healthcare professional’s assistance. This study’s objective is to investigate the spatial variation and factors associated with home delivery among reproductive-age women in rural Ethiopia.
Methods
The study used data from the Ethiopian Mini Demographic and Health Survey 2019. A weighted sample of 4160 reproductive-age women was employed in this study. For spatial analysis, Global Moran’s I and Getis-Ord Gi* analysis was used to detect the presence of clustering and hotspot areas of home delivery respectively. A multilevel logistic regression model was employed to identify factors associated with home delivery. An adjusted odds ratio with a corresponding 95% confidence interval and a p-value of < 0.05 were used to declare statistical significance.
Results
The prevalence of home delivery in rural Ethiopia was 58.72% (95% CI: 57.20, 60.20). The spatial analysis showed the spatial disparities of home delivery across rural Ethiopia (global Moran’s I = 0.525, p-value=0.042). Getis-Ord Gi* analysis identified significant hotspot areas of home delivery in the Somali, Afar, Harari, and Dire Dawa regions. Rich wealth index (AOR = 0.392, 95% CI: 0.316, 0.487), secondary and above education (AOR = 0.297, 95% CI: 0.202, 0.438), household family size 7 and above (AOR= 2.279, 95% CI:1.643, 3.161), multiple births (AOR = 0.429, 95% CI: 0.269, 0.685), community poverty (AOR= 2.084, 95% CI:1.712,2.538) and community illiteracy (AOR= 2.232, 95% CI:1.864,2.674) were significant determinant factors for home delivery.
Conclusion
The proportion of home delivery in rural Ethiopia is still more than fifty percent and the study showed significant spatial disparities across the regions of Ethiopia. Mother’s educational level, parity, religion, family size, type of birth, wealth index, community poverty, and community illiteracy were found to be significant determinants of home delivery. Moreover, a health promotion strategy and public awareness to scale up education, and improving economic status are vital to reduce home delivery in the identified hotspot areas. Considering the finding that the education of women affects the place of delivery, the Ethiopian government with stakeholders should enhance initiatives to improve the educational status of women. Moreover, planners and policymakers should make further efforts to alleviate poverty at the household and community level.
1. INTRODUCTION
Childbirth is a complex process, and it is crucial to ensure that all necessary services are given to guarantee the safest care for both mother and newborn [1]. Maternal mortality remains a global public health issue, with 303,000 women dying each year due to childbirth-related complications [2]. In low-income nations, parental deaths accounted for 99% of all deaths. Among these, Sub-Saharan Africa accounts for 66% of maternal deaths [3]. Ethiopia has one of the highest rates of maternal death worldwide [4].
The World Health Organization (WHO) recommends that every birth should be attended by a skilled birth attendant. However, a large proportion of deliveries occur at home without the support of health professionals [5]. The majority of maternal deaths result from direct obstetric causes, including postpartum hemorrhage, miscarriage, obstructed labor, pregnancy-induced hypertension, fetal asphyxia, and stillbirth. These conditions can be effectively managed to reduce the number of maternal deaths by having skilled birth attendants perform during the delivery in a health facility [6, 7].
Although maternal mortality has decreased significantly in high-income countries [8], Ethiopia still has a very high rate with 412 deaths per 100,000 live births, nearly twice the global average of 211 deaths per 100,000 live births [9, 10] and skilled birth attendants have the potential to prevent over 75% of maternal birth-related deaths [11].
Home delivery is still a common public problem across Ethiopia. However, the country must work hard to achieve the ambitious Sustainable Development Goal (SDG) 2030 target of 70/100,000 maternal deaths and the Health Sector Transformation Plan (HSTP) target of 199/100,000 live births MMR in 2020 [12]. Compared to urban areas, the prevalence of home delivery is more common in rural residential areas (59%) than urban (39%) [13].
The risk of infection, postpartum hemorrhage, and the spread of HIV/AIDS are all increased when giving birth at home. The burden of home delivery, which is primarily unsupervised, leads to morbidity and mortality among children as well as issues with maternal health [14].
Numerous studies have examined the prevalence and spatial distribution of home and institutional deliveries among women of reproductive age. However, a detailed analysis of the spatial distribution, including hot spots and cold spots, geographic patterns, and spatial dependencies of home deliveries among women in rural Ethiopia, has not yet been explored using current DHS data [15-20]. To better understand the factors associated with home delivery in rural Ethiopia, it is necessary to incorporate the hierarchical structure of population data. Addressing regional disparities in access to maternal health care services should be a priority for reducing maternal mortality in rural Ethiopia. Additionally, the target groups should be identified for specific interventions using appropriate statistical methods. To such a literature gap, we conducted a spatial and multilevel analysis to identify factors associated with home delivery among reproductive-aged women in rural Ethiopia using the 2019 Ethiopian Mini Demographic and Health Survey data. Therefore, detecting the geographic variation of home delivery among reproductive-aged women is important to prioritize and design targeted intervention programs to reduce home delivery, especially in those areas with a higher risk of home delivery in rural communities that improves maternal and neonatal health outcomes. Moreover, this study will help policymakers, program planners, and other stakeholders in guiding health investment and developing prevention and intervention programs.
2. DATA AND METHODS
2.1. Study Area and Data Source
This current study is conducted in Ethiopia which is divided into nine administrative regions (Afar, Amhara, Benishangul-Gumuz, Gambella, Harari, Oromia, Somalia, Southern Nations Nationalities and People’s Region, and Tigray); Addis Ababa and Dire Dawa administrative cities [21, 22]. The study employed secondary data from the 2019 Ethiopian Mini Demographic and Health Survey.
2.2. Population and Sampling Procedure
A two-stage stratified cluster sampling was used. Each region was divided into urban and rural areas. First, a total of 305 enumeration areas (EAs) (93 in urban, 212 in rural) were chosen independently with a probability proportional to each EAs. Second, a fixed number of 30 households/clusters were selected with an equal probability of systematic selection. The detailed sampling procedures are available on the measure DHS website in the 2019 EMDHS report (https://www.dhsprogram.com). For this study, the individual record (IR) data set was used. The source population for this study was all reproductive age group women (15–49) in rural Ethiopia. The study participants were a 4160 weighted sample of reproductive-age women living in rural Ethiopia who gave birth in the last 5 years preceding the survey for the recent birth.
2.3. Study Variable
The response variable was the place of delivery of the mother which is binary and is coded as 1 if they had a birth at home and 0 if they had a birth at health institution. In this study, both individual-level and community-level factors were included as independent variables. Individual level variables were the marital status of the mother, religion of the mother, types of birth, wealth index of family, mother’s education level, age of mother at the first birth, the current age of the mother, birth order, family size, mass media exposure, sex of household head and parity and community-level variables were community poverty, community education and region were included in this study. In EMDHS data, there was no variable collected at the community level except region. Therefore, we generated community-level variables at the cluster level.
In this study, mass media exposure was considered by combining the access of television or radio or both and it was categorized as “exposed”. But, if they do not have any exposure from these outlets we coded it as “no media exposure” [1]. This study considers two community-level factors: community literacy and poverty index. The first factor, community-level literacy, is defined by the proportion of mothers within the cluster who attended primary, secondary, or higher education. The second community-level factor is the poverty index, which reflects the proportion of poor mothers within the cluster; this proportion is aggregated to indicate the overall poverty status within the cluster [1].
2.4. Data Management and Analysis
Descriptive statistics and analytical analysis were performed using STATA version 14 statistical software. Sampling weight was applied for this complex survey design using primary sampling unit, strata, and women’s weight (V005) to compensate for the disproportional probability of sampling and non-response rate between the strata that have been geographically defined [23].
2.5. Spatial Analysis
ArcGIS version 10.8 was used for exploring the spatial distribution, global spatial autocorrelation, and spatial interpolation for identifying significant hotspot areas of home delivery among reproductive age women in rural Ethiopia [24].
2.6. Spatial Autocorrelation Analysis
The spatial autocorrelation (Global Moran’s I) statistic was used to evaluate whether the home delivery patterns were dispersed, clustered, or randomly distributed during the survey periods in rural Ethiopia. When the Moran’s I value is close to -1 indicates home delivery is dispersed, whereas Moran’s I close to +1 indicates home delivery is clustered in the study area. However, Moran’s I value zero shows a random distribution of home delivery [25]. A statistically significant Moran’s I (p-value<0.05) asserts the presence of spatial autocorrelation as well as it detects the existence of at least one cluster, but not the specific location of the cluster(s) [1].
The Getis-Ord Gi* hot spot analysis was used to identify hot spots and cold spots of home delivery among reproductive age women in rural Ethiopia. It helps to classify autocorrelation into negative or positive autocorrelation [26]. A Z-score near zero indicates perfect randomness and a positive Z-score indicates statistical clustering of the hotspots of home delivery. However, a negative z-score indicates a statistical clustering of home births with low cold spots among reproductive women in rural Ethiopia. Areas at high risk (hotspot) of home delivery with high Gi* and areas at low risk (cold spot) of home delivery with low Gi* were detected [27].
2.7. Spatial Interpolation
This technique was applied to predict home delivery for the un-sampled areas based on sampled clusters using the geo-statistical Ordinary Kriging spatial interpolation method [19]. The interpolation was performed based on the assumption that spatially distributed objects are spatially correlated; in other words, things that are close together tend to have similar characteristics [28]. Therefore, a smooth surface for the risk areas of home delivery among reproductive women was indicated on the home delivery risk map [29].
2.8. Multilevel Logistic Regression Analysis
Multilevel models were developed to overcome the analytical problems that arise when data are hierarchically organized, and sampled data are a sample of several stages of this hierarchical nature of data. EMDHS was a two-stage stratified cluster sampling design that followed a hierarchical sampling technique. Multilevel models account for the interdependence of observations within the same group, ensuring more accurate estimates of the effects of individual and contextual factors. To estimate both fixed effects and random effects on home delivery, a two-level mixed-effect logistic regression model was employed [30]. The individual and community level variables which were statistically significant at p-value 0.25 in the bi-variable multilevel mixed-effects logistic regression analysis were considered for the final individual and community level model adjustments. In the multivariable multilevel analysis, variables with a p-value of less than 0.05 were considered statistically significant [31].
2.9. Model Building
Model I (Null model) was fitted without explanatory variables to test random variability and to estimate the intra-class correlation coefficient, and Proportional Change in Variance (PCV) [32]. Model II examined the effects of individual-level characteristics, Model III examined the effect of community-level factors, and Model IV examined the effects of both individual and community-level characteristics simultaneously. For measurements of variation (random effects), intra-class correlation coefficient (ICC) [32], Median Odds Ratio (MOR) [33], and proportional change in variance (PCV) statistics were computed. Model comparisons were assessed based on the Likelihood Ratio (LR) test and different Information Criteria [34].
The intra-class correlation indicates the proportion of the variance explained by the grouping structure in the population. When the logistic regression model is used the residual at level one (women level) is assumed to follow the standard logistic distribution with mean 0 and variance
and it is expressed as:
, where
is the variance of the higher level (region).
The aim of the median odds ratio [33] is to translate the area level variance in the widely used odds ratio (OR) scale, which has a consistent and intuitive interpretation. The MOR is defined as the median value of the odds ratio between the area at the highest risk and the area at the lowest risk when randomly picking out two areas. The MOR can be conceptualized as the increased risk that (in median) would have if moving to another area with a higher risk. It is computed by [35]
![]() |
where;
is the area level variance, and 0.6745 is the 75th percentile of the cumulative distribution function of the standard normal distribution with mean 0 and variance 1. Whereas the proportional change in variance is calculated as [36].
![]() |
where; VA = variance of the initial model, and VB = variance of the model with more factors.
The model's fit was assessed using AIC and BIC, and results were presented as adjusted odds ratios to facilitate interpretation.
3. RESULTS
In this study, a total of 4160 weighted sample reproductive-age women who gave birth within five years preceding the survey were included. The prevalence of home delivery in rural Ethiopia was 58.72% (95% CI: 57.20, 60.20) (Table 1).
- | Proportion | Std.Err. | 95%CI | |
---|---|---|---|---|
Place of delivery | Health facility | 0.413 | 0.008 | 0.398, 0.428 |
Home | 0.587 | 0.008 | 0.572, 0.602 |
Among the total studied women, 70.8% were aged group 21–34 years and 55.9% were from poor households. More than half of women (57.2%) of the women space their children less than 19 months from this (34.8) % gave birth at home and (42.8%) of the women space their children 19 and above months from this (23.9%) gave birth at home. The majority of respondents (76.2%) were did not access media in their household from this 47.5% given birth at home. More than half (60.5%) of women had not attend formal education, out of this (41.9%) of women deliver their child at home. From the regions in Ethiopia, 26.4% from Oromia, 10.4% from Amhara, and 11.3% from SNNPR of women deliver their child at home in rural Ethiopia. From community literacy level about 40.9% of community is below the national median literacy level out of this 30.7% women gave births at home (Table 2).
Variables | Categories | Place of Delivery | |||||
---|---|---|---|---|---|---|---|
Health Institution | Home | Total | |||||
Frequency | % | Frequency | % | frequency | % | ||
Region | Tigray | 168 | 4.0 | 97 | 2.3 | 265 | 6.4 |
Afar | 10 | 0.2 | 53 | 1.3 | 63 | 1.5 | |
Amhara | 385 | 9.3 | 437 | 10.5 | 822 | 19.8 | |
Oromia | 724 | 17.4 | 1099 | 26.4 | 1823 | 43.8 | |
Somali | 36 | 0.9 | 252 | 6.1 | 288 | 6.9 | |
Benshangul-gumuz | 30 | 0.7 | 17 | 0.4 | 47 | 1.1 | |
SNNPR | 346 | 8.3 | 468 | 11.3 | 814 | 19.6 | |
Gambela | 5 | 0.1 | 6 | 0.1 | 11 | 0.3 | |
Harari | 4 | 0.1 | 5 | 0.1 | 9 | 0.2 | |
Dire Dawa | 8 | 0.2 | 8 | 0.2 | 16 | 0.4 | |
Current age of mother | 20 and less | 115 | 2.8 | 92 | 2.2 | 207 | 5.0 |
21-34 | 1247 | 30.0 | 1698 | 40.8 | 2945 | 70.8 | |
35-49 | 355 | 8.5 | 653 | 15.7 | 1008 | 24.2 | |
Religion of mother | Orthodox | 662 | 15.9 | 705 | 16.9 | 1367 | 32.9 |
Protestant | 424 | 10.2 | 603 | 14.5 | 1027 | 24.7 | |
Muslim | 614 | 14.8 | 1054 | 25.3 | 1668 | 40.1 | |
Others | 17 | 0.4 | 81 | 1.9 | 98 | 2.4 | |
Types of birth | Single | 1665 | 40.0 | 2398 | 57.6 | 4063 | 97.7 |
Multiple | 52 | 1.3 | 45 | 1.1 | 97 | 2.3 | |
Marital status | Unmarried | 89 | 2.1 | 127 | 3.1 | 216 | 5.2 |
Married | 1628 | 39.1 | 2315 | 55.7 | 3943 | 94.8 | |
Wealth index of house hold | Poor | 669 | 16.1 | 1655 | 39.8 | 2324 | 55.9 |
Medium | 448 | 10.8 | 513 | 12.3 | 961 | 23.1 | |
Rich | 600 | 14.4 | 275 | 6.6 | 875 | 21.0 | |
Mother’s Education level | No education | 775 | 18.6 | 1743 | 41.9 | 2518 | 60.5 |
Primary | 740 | 17.8 | 655 | 15.7 | 1395 | 33.5 | |
Secondary and above | 202 | 4.9 | 45 | 1.1 | 247 | 5.9 | |
Family size | Less than 4 | 290 | 7.0 | 128 | 3.1 | 418 | 10.1 |
4-6 | 917 | 22.0 | 1188 | 28.6 | 2105 | 50.6 | |
7 and above | 509 | 12.2 | 1127 | 27.1 | 1636 | 39.3 | |
Age mother at first birth | Less than 19 | 932 | 22.4 | 1148 | 34.8 | 2380 | 57.2 |
19 and above | 785 | 18.9 | 995 | 23.9 | 1780 | 42.8 | |
Birth order | First | 505 | 12.1 | 310 | 7.5 | 815 | 19.6 |
2-3 | 521 | 12.5 | 694 | 16.7 | 1215 | 29.2 | |
4-5 | 361 | 8.7 | 675 | 16.2 | 1036 | 24.9 | |
6 and above | 330 | 7.9 | 763 | 18.3 | 1093 | 26.3 | |
Sex of household head | Male | 1545 | 37.1 | 2162 | 52.0 | 3707 | 89.1 |
Female | 172 | 4.1 | 280 | 6.7 | 452 | 10.9 | |
Media exposure | No | 1190 | 28.6 | 1978 | 47.5 | 3168 | 76.2 |
Yes | 527 | 12.7 | 465 | 11.2 | 992 | 23.8 | |
Parity | 2 and less | 759 | 18.2 | 520 | 12.5 | 1279 | 30.7 |
3-5 | 580 | 13.9 | 1019 | 24.5 | 1599 | 38.4 | |
6 and above | 378 | 9.1 | 904 | 21.7 | 1282 | 30.8 | |
Community poverty | Low | 1400 | 33.7 | 1296 | 31.2 | 2696 | 64.8 |
High | 317 | 7.6 | 1147 | 27.6 | 1464 | 35.2 | |
Community literacy | Low | 1292 | 31.1 | 1167 | 28.1 | 2459 | 59.1 |
High | 425 | 10.2 | 1276 | 30.7 | 1701 | 40.9 |
3.1. Result of Spatial Analysis
3.1.1. Spatial Autocorrelation of Home Delivery
The estimated Moran’s index value was equal to 0.523716 (p-value=0.042) which was positive and indicated that the distribution of home delivery was significantly clustered between rural areas of regions of the country (p-value<0.05) (Fig. 1).
3.1.2. Hotspot Analysis
In EMDHS-2019 sampled data; the rural area of Somali, Afar, Harari and Dire Dawa were identified as hot spot (high risk) regions for home delivery. In contrast, the rural area of Amhara region was identified as cold spot (low risk) regions (Fig. 2).
In the interpolation analysis, the highest prevalence of home delivery was detected in Somali, Afar, Harari, Dire Dawa, Oromia and southern nations nationalities and people regions of rural areas. In contrast, the predicted low prevalence of home delivery was identified in Benishangul-Gumaz, Tigray, Amhara, and Gambela regions of rural areas (Fig. 3).
Kriging spatial interpolation revealed that; Northeast and Southeast of Somali, South and North parts of Oromia, and south part of Afar, Harari and Dire Dawa regions of rural areas have a high proportion of home delivery, in contrast in Tigray, in most parts of Amhara, in some parts of Gambela, Beshangl Gumz and weast Oromia region of rural area have low proportion of home delivery (Fig. 4).
3.1.3. Multilevel Logistic Regression Analysis
The variables age of the mother, wealth index, mother’s educational level, religion, type of birth, family size, age at first birth, birth order, media exposure, parity, community education, and community poverty were included in a multivariable analysis. However, current marital status (p-value=0.715) and household head sex (p-value=0.287) were excluded at p< 0.25 (Table 3).
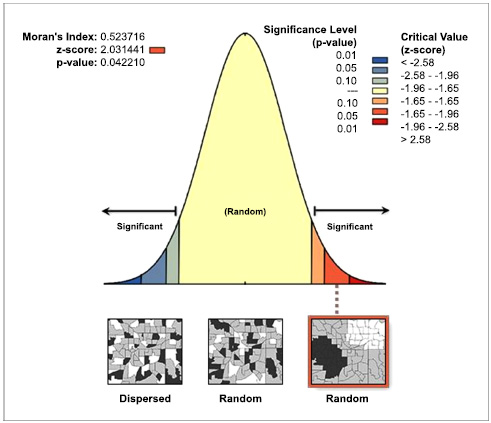
A spatial autocorrelation analysis (Moran’s I) of home delivery in rural areas of Ethiopia based on EMDHS 2019.
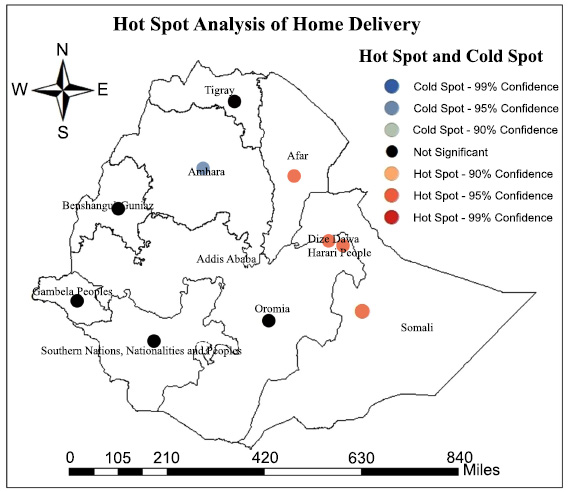
Hot spot analysis of home delivery in rural areas of Ethiopia based on the 2019 EMDHS.
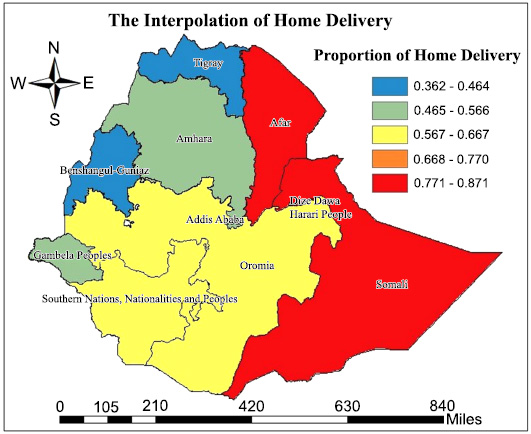
Spatial interpolation of home delivery in Ethiopia in EMDHS in 2019.
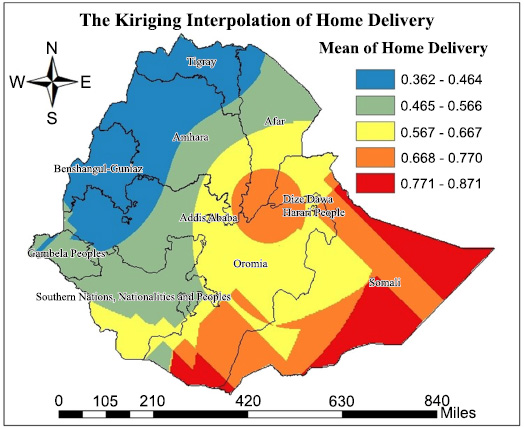
Kiriging interpolation of home delivery in Ethiopia in EMDHS in 2019.
Variables | Category | COR | p-value | 95% CI |
---|---|---|---|---|
- | 20 and less (Ref.) | - | - | - |
Age of mother | 21-34 | 1.828 | ≤0.001* | 1.364, 2.452 |
35-49 | 2.591 | ≤0.001* | 1.893, 3.546 | |
Religion | Orthodox(Ref) | - | - | - |
Protestant | 1.018 | 0.881 | 0.810, 1.278 | |
Muslim | 0.927 | 0.433 | 0.737, 1.139 | |
Others | 3.356 | ≤0.001* | 1.925, 5.845 | |
Type of birth | Single(Ref.) | - | - | - |
Multiple | 0.520 | 0.002** | 0.342, 0.790 | |
Wealth index | Poor(Ref.) | - | - | - |
Medium | 0.510 | ≤0.001* | 0.435, 0.598 | |
Rich | 0.203 | ≤0.001* | 0.171, 0.241 | |
Mothers education level | No education(Ref) | - | - | - |
Primary | 0.390 | ≤0.001* | 0.339, 0.449 | |
Sec. and above | 0.099 | ≤0.001* | 0.071, 0.140 | |
Family size | 1-3 (Ref.) | - | - | - |
4-6 | 3.077 | ≤0.001* | 2.442, 3.877 | |
7 and above | 4.885 | ≤0.001* | 3.845, 6.206 | |
Age at first birth | Less than 19 (Ref.) | - | - | - |
19 and above | 0.820 | 0.002* | 0.722, 0.932 | |
Birth order | First (Ref.) | - | - | - |
2-3 | 2.211 | ≤0.001* | 1.835, 2.663 | |
4-5 | 3.121 | ≤0.001* | 2.567, 3.795 | |
6 and above | 2.697 | ≤0.001* | 3.039, 4.498 | |
Household head sex | Male(Ref.) | - | - | - |
Female | 0.889 | 0.287 | 0.715, 1.104 | |
Media exposure | No(Ref.) | - | - | - |
Yes | 0.555 | ≤0.001* | 0.478, 0.643 | |
Marital status | Unmarried (Ref.) | - | - | - |
Madrid | 0.948 | 0.715 | 0.714, 1.261 | |
Parity | 2 and less(Ref.) | - | - | - |
3-5 | 2.610 | ≤0.001* | 2.234, 3.048 | |
6 and above | 3.334 | ≤0.001* | 2.821, 3.944 | |
Community poverty | Low(Ref.) | - | - | - |
High | 1.326 | ≤0.001* | 1.167, 1.485 | |
Community education | Low(Ref.) | - | - | - |
High | 1.401 | ≤0.001* | 1.244, 1.557 |
Parameters | Null Model | Model II | Model III | Model IV | |
---|---|---|---|---|---|
Region -level variance (SE) | 0.680 (0 .353) | 0.619(0.334) | 0.519(0.284) | 0.487(0.289) | |
ICC (%) | 17.11% | 15.83% | 13.63% | 12.89% | |
PCV (%) | Reference | 8.97% | 23.68% | 28.38% | |
MOR | 2.195 | 2.118 | 1.988 | 1.955 | |
Model fit statistics | |||||
Log-likelihood | -2741.709 | -2380. 162 | -2499.977 | -2293.327 | |
AIC | 5487.420 | 4802.323 | 5007.953 | 4632.657 | |
BIC | 5500.210 | 4936.619 | 5033.533 | 4779.743 |
3.2. Result of Multilevel Logistic Regression Analysis
Table 4 shows the result of the multilevel logistic regression analysis. The null model (model I) revealed there is considerable variation in the odds of home delivery across regions (ICC=17.11% which indicates that 17.11% of the variation in the home delivery was attributed to regions).
In model II, only individual-level variables were added. The ICC in Model II indicated that 15.83% of the variation in women’s home delivery was attributable to differences across regions. As shown by the PCV, 8.97% of the variance in home delivery across regions was explained by the individual-level characteristics.
In model III, only community-level variables were added. About 23.68% of the variation in the odds of home delivery was attributed to community-level factors (PCV =23.68%), and 13.63% of the variation in home delivery was attributed to regional factors (ICC = 13.63%).
In model IV, both individual-level and community-level variables were included and the estimated ICC, about 12.89% of the variability among communities in-home delivery was due to regional factors. The PCV indicated that 28.38% of the variation in home delivery across communities was attributed to both individual and community-level factors.
Including both individual and community-level factors reduced the unexplained heterogeneity in-home delivery between communities from a MOR of 2.195 in the null model to an MOR of 1.955 in the final model. The probability of home delivery increases by 0.24 times for women who relocated from low-risk to high-risk communities (Table 4).
3.3. Model Fit Statistics
As it is shown in the table, the model with the smallest AIC and BIC (model IV) exhibited which is suited to the data. For both the individual and community-level variables, model IV was modified. As a result, the covariates mother’s education level, wealth index of household, family size, type of birth, parity, religion of mother, and community poverty were statistically significantly associated with home delivery in rural areas of Ethiopia.
Model IV, the final model included both the individual and community-level characteristics simultaneously. The likelihood of home delivery for protestant and other religious follower was increased by 55.3% (AOR= 1.553, 95% CI: 1.183, 1.986) and 65.1% (AOR =2.651, 95% CI: 1.455, 4.831) compared to orthodox religion follower respectively.
Women who had primary education were 31.3% (AOR = 0.687: 95% CI: 0.561, 0.707) less likely to give birth at home as compared to those who had no education. Similarly, Women who had secondary and above education were 70.3% (AOR = 0.297: 95% CI: 0.202, 0.438) less likely to give birth at home as compared to women who had no education.
The odds of home delivery among women with rich wealth status were 60.8% (AOR =0.392, 95% CI: 0.316, 0.487) less likely to give birth at home as compared to poor wealth status households of rural Ethiopia.
The odds of home delivery among the women who had multiple births were 0.392 times (AOR = 0.392; 95% CI: 0.316, 0.487) less likely to have home delivery as compared to those who had single birth.
The odds of home delivery among the women who had parity of 3-5 were 1.548 times (AOR = 1.548; 95% CI: 1.195–1.986), 6 and above were 1.676 times (AOR = 1.676; 95% CI: 1.035-2.715) more likely to deliver home compared to women who had 2 and less.
Women living in communities with a high proportion of poverty were 2.084 times (AOR = 2.084; 95% CI: 1.712-2.538) more likely to give birth at home compared to women living in communities with a low proportion of poverty (Table 5).
4. DISCUSSIONS
This study aimed to explore the spatial distribution and associated factors of home deliveries among reproductive-age women in rural Ethiopia. The prevalence of home delivery in this study was 58.72% (95% CI: 57.22 – 60.21) in 2019. This finding was higher than that of the study conducted in Sub-Saharan African countries which was 44% [37] and rural communities of Eritrea which was 75.4% [5]. It was less than the result from a study done in Afar, Ethiopia (71%) [38]. This discrepancy might be due to the study setting, time variation, sample size difference, women’s attitudes, and cultural differences could be the plausible reasons. Across regions, there was a variation in home delivery among reproductive-age women in rural Ethiopia.
The multilevel logistic regression analysis confirmed the significance of the regional difference in home delivery in rural Ethiopia which accounts for about 17% of the entire variation in home delivery.
The result from multilevel logistic regression analysis showed that the mother’s education level, wealth index of household, family size, type of birth, parity, mother’s religion, and community poverty were the predictors of home delivery in rural Ethiopia at 95% level of significance.
The wealth index of households was significantly associated with home delivery. Women who lived in rich wealth index households had 0.392 times less likely to have a home delivery as compared with those who lived in poor wealth index households. This finding is consistent with studies conducted in Ethiopia and Eritrea [5, 39, 40]. This might be due to the lack of access to health care, transportation, and other costs that women can afford to pay such fees are more likely to seek medical help. Ethiopia provides free maternity and ambulance services. Nonetheless, since there aren't many pharmaceuticals available in health institutions and very few ambulances, medication and transportation services are still paid for out of pocket.
Variables | Category | AOR | p-value | 95% CI AOR |
---|---|---|---|---|
- | 20 and less (Ref) | 1.00 | - | - |
Age of mother | 21-34 | 0.998 | 0.992 | (0.687, 1.449) |
35-49 | 0.840 | 0.444 | (0.537, 1.313) | |
Religion | Orthodox (Ref) | 1.00 | - | - |
Protestant | 1.533 | <0.001* | (1.183, 1.986) | |
Muslim | 0.774 | 0.046* | (0.601, 0,996) | |
Others | 2.651 | <0.001* | (1.455, 4.831) | |
Type of birth | Single(Ref) | 1.00 | - | - |
Multiple | 0.429 | <0.001* | (0.269, 0.685) | |
Wealth index | Poor(Ref) | 1.00 | - | - |
Medium | 0. 849 | 0.091 | (0.701, 1.027) | |
Rich | 0.392 | <0.001* | (0.316, 0.487) | |
Mothers education | No education(Ref) | 1.00 | - | - |
Primary | 0.687 | <0.001* | (0.561, 0.797) | |
Secondary and above | 0.297 | <0.001* | (0.202, 0.438) | |
Family size | 1-3 (Ref) | 1.00 | - | - |
4-6 | 1.792 | <0.001* | (1.344, 2.391) | |
7 and above | 2.279 | <0.001* | (1.643, 3.161) | |
Age at first birth | Less than 19 (Ref) | 1.00 | - | - |
19 and above | 1.078 | 0.372 | (0.915, 1.269) | |
Birth order | First (Ref) | 1.00 | - | - |
2-3 | 1.215 | 0.148 | (0.933, 1.581) | |
4-5 | 1.138 | 0.473 | (0.800, 1.619) | |
6 and above | 1.106 | 0.716 | (0.643, 1.901) | |
Media exposure | No(Ref) | 1.00 | - | - |
Yes | 1.064 | 0.508 | (0.886, 1.278) | |
Parity | 2 and less(Ref) | 1.00 | - | - |
3-5 | 1.548 | 0.001* | (1.195, 1.986) | |
6 and above | 1.676 | 0.036* | (1.035, 2.715) | |
Community poverty | Low(Ref) | 1.00 | - | - |
High | 2.084 | <0.001* | (1.712,2.538) | |
Community education | Low(Ref) | 1.00 | - | - |
High | 2.232 | <0.001* | (1.864,2.674) |
The study also shows that women’s education level is crucial in determining their place of birth. Women who didn’t attain formal education had a higher likelihood of home delivery than women who had formal education, which is consistent with previous studies [14, 39-44]. The possible explanation could be that educated women have enough knowledge of giving birth, going to medical facilities, being financially independent, and accepting health professionals' advice.
The odds of home delivery for mothers having multiple births were less likely as compared to those having single births. This study is supported by [45]. The possible explanations for this result may be the increased number of pregnancies and the complexity of pregnancies may be the causes for compelling women to visit a medical facility in order to give birth safely.
In addition, home birth opportunities for mothers who are Muslim followers were less than for mothers who follow the Orthodox faith. This finding was contrary to other related studies that showed that religion influenced a mother’s choice of delivery [39]. The main reason for this may be those conventional gives further education about giving birth at home in addition to theological parts.
In this study, family size was also a predictor of home delivery in rural areas of Ethiopia. The odds of home delivery for women with family size 7 and above and 4-6 were more likely compared to women with family size 1-3. This result is in line with a study carried out in southwest Ethiopia [46]. This is since mothers with large families lack the resources to seek out a health facility for childbirth and may not recognize the importance of institutional delivery.
The spatial analysis realized that there is significant geographic variation in home delivery in rural areas of Ethiopia. Hot spot locations with high home delivery were identified in the Somali, Afar, Harari, and Dire Dawa regions of rural areas observed. This is due to the poor accessibility of infrastructure such as roads for transportation and ambulances [47]. Furthermore, these communities were more pastoral areas; as a consequence, relative to the rest, poor access to education, and were not permanent residents because in these areas, there is limited availability and accessibility of maternal health services [48].
4.1. Strengths and Limitations of the Study
The data for this study was extracted from the Ethiopian Mini Demographic and Health Survey, which is a large data set and covers a large geographical area. Furthermore, combinations of statistical methods (spatial analysis and multilevel modeling) approach were applied for this study that allow the clustering effect as the best approximation to quantify the heterogeneity in the home delivery. The limitation of the study was a cross-sectional survey, which may not help establish a temporal relationship between the independent variable and dependent variable. The data was collected by interviewing the women; this may result in a possibility of recall and social desirability biases that will result in underreporting and misreporting of events. Moreover, this survey is a mini survey that lacks some important variables such as occupational status, the distance to health facility, health insurance coverage, and others.
CONCLUSION
In rural Ethiopia, the prevalence of home delivery is spatially varied among regions. Some regions had a high proportion of home delivery. The highest prevalence of home delivery was detected in Somali, Afar, Harari, and Dire Dawa regions of rural areas. Among the study covariates mothers’ education level, mothers’ religion, wealth index of household, family size, type of birth, parity, community education, and community poverty were significant predictors of home delivery among reproductive-age women in rural Ethiopia. The government and public health institutions should provide a variety of care to reduce giving birth at home among uneducated women, poor women, and women living in hot spot areas (Afar, Harri, Dire Dawa, and Somali). Strategies should be developed to expand access to institutional delivery services among women living in rural Ethiopia. It is also better to consider the individual, and community-level risk factors that may help planners, policymakers, healthcare providers, and decision-makers emphasize institutional delivery.
Moreover, target information for vulnerable groups like women in rural areas, launching awareness campaigns about the benefits of institutional delivery.
Implications for Policy Practice and Further Research
Considering the finding that the education of women affects the place of delivery, the Ethiopian government should develop initiatives to improve the educational status of women. Moreover, planners and policymakers should make further efforts to alleviate poverty at the household and community level. Since community-level illiteracy showed a significant effect on women’s experience of home delivery, the Ministry of Health should work in collaboration with the Ministry of Education to improve the literacy status of women in the community. In addition, cultural beliefs related to home delivery should be investigated. Moreover, the inclusion of qualitative data could add depth to the analysis, offering insights into the lived experiences of women who choose home delivery. Policymakers should focus on improving access to health facilities to improve family planning service utilization and reduce home delivery.
AUTHORS' CONTRIBUTIONS
D.B.B., K.T., N.A.B., and M.T.: Involved in the conception, design, acquisition, analysis, and interpretation of data; D.C.: Involved in a thorough revision of essential intellectual content. The article was read and approved by every author.
LIST OF ABBREVIATIONS
AOR | = Adjusted odds ratio |
CI | = Confidence Interval |
DHS | = Demographic and health survey |
EMDHS | = Ethiopian mini demographic and health survey |
ICC | = Intra-class correlation coefficient |
MOR | = Median odds ratio |
PCV | = Proportional change in variance |
SSA | = Sub-Saharan Africa |
WHO | = World Health Organization |
HSTP | = Health Sector Transformation Plan |
SDG | = Sustainable Development Goal |
IR | = Individual Record |
AVAILABILITY OF DATA AND MATERIALS
The data supporting the findings of the article is available in the Demographic and Health Surveys (DHS) Program at: https://www.dhsprogram.com/Data/.
FUNDING
This work is partially based upon research supported by the South Africa National Research Foundation (NRF) and South Africa Medical Research Council (SAMRC) (South Africa DSTNRF-SAMRC SARCHI Research Chair in Biostatistics, Grant number 114613). Opinions expressed and conclusions arrived at are those of the author and are not necessarily to be attributed to the NRF and SAMRC.
ACKNOWLEDGEMENTS
We would like to thank the DHS program, for providing the dataset used in this study.