All published articles of this journal are available on ScienceDirect.
Assessing the Impact of Artificial Intelligence Applications on Diagnostic Accuracy in Saudi Arabian Healthcare: A Systematic Review
Abstract
Background
Artificial intelligence (AI) has become a disruptive force with great potential to revolutionize healthcare. The integration of artificial intelligence in healthcare practices and its use in areas, such as the detection and diagnosis of diseases, has led to an increased interest in this topic, which has been key in informing this research study to determine its effect on diagnostic accuracy. However, the impact of AI on healthcare diagnostic accuracy, particularly in the context of Saudi Arabia, remains an underexplored area of research.
Aim
This systematic review sought to address this gap by analyzing the effect of AI applications' diagnostic accuracy in Saudi Arabia's healthcare.
Methods
The study employed a structured and systematic search strategy in compliance with the Preferred Reporting Items for Systematic Reviews and Meta-Analyses (PRISMA) criteria. Three databases were used to identify the articles, including PubMed, Embase, and CINAHL. The search for relevant articles linked to the impact of AI applications on diagnostic accuracy in the KSA healthcare sector was narrowed down to articles published between 2013 and 2023. This step generated 450 articles, which were further evaluated based on the inclusion criteria of the study to narrow down to 12 articles for analysis.
Results
11 out of 12 studies were conducted between 2020 and 2023, indicating that the last three years have witnessed the largest number of studies on artificial intelligence. The included studies were conducted in KSA and within different hospitals. The studies included 7 cross-sectional studies, 3 observational studies (1 retrospective study), 1 experimental study, and 1 randomized controlled trial (RCT). They all showed that the use of AI has been increasing in healthcare, and its use is enhancing the overall healthcare outcomes and is helpful in a wide variety of diseases and conditions, including chronic diseases.
Conclusion
The models have shown that the use of AI is capable of enhancing diagnostics and treatment quality, which can be essential in planning for preventing care in line with Vision 2030. Hence, the findings of this systematic review can contribute to a better understanding of the role of AI applications in diagnostic accuracy within Saudi Arabia's healthcare and offer insights applicable to regions facing similar challenges.
1. INTRODUCTION
The healthcare landscape is rapidly evolving and just like any other sector of the global economy, artificial intelligence (AI) has become a disruptive force with great potential to revolutionize healthcare. Overall, the advancements in technology have had a positive effect on the overall healthcare sector and resulted in exponential development in this area with regard to materials used, diagnosis, and treatment [1]. Hence, the integration of artificial intelligence in healthcare practices and its use in areas, such as the detection and diagnosis of diseases, has led to an increased interest in this topic, and this has been key in informing this research study to determine its effect on diagnostic accuracy.
Despite the high burden of diseases that countries are experiencing and the need to enhance accurate and timely diagnosis, multiple challenges hinder the provision of optimal services to patients. The lack of resources, availability of skills and expertise, high costs, etc., can be overcome by investing an amount of time in the sector to meet the job demands [2]. Progress has been made in Saudi Arabia to enhance these skills in line with Vision 2030, but the capacity is still lagging. As noted in the research work conducted by Zanella-Calzada et al. [3], the progress of diagnosis in many nations has always been a challenge as the whole process is complex and requires doctors and physicians to analyze comprehensive data, medical images, and history. However, innovation and technology have proven to play a critical role in trying to overcome this. The introduction of artificial intelligence in the healthcare setting has led to innovation in the diagnosis of patients, management, and prognosis of health-related conditions at a faster pace. The preliminary review has shown that AI has the potential to enhance diagnostic accuracy, help medical professionals reduce errors, and enhance efficiency, hence improving overall medical outcomes and implying the potential of improving Saudi Arabia's healthcare to meet Vision 2030 protocols. The KSA Vision 2030 has taken center stage in developing its healthcare sector, whereby it is seeking to transform the healthcare system into a comprehensive, effective, and integrated system. As noted by Alasiri and Mohammed [4], this enhanced system prioritizes innovation and disease prevention while enhancing access to quality care. Hence, the integration of AI in Saudi Arabia's healthcare might be key to enhancing the quality of care by improving diagnostic accuracy and, hence, transforming patient care and outcomes.
The advances in digital healthcare technologies, especially artificial intelligence, robotics, nanotechnology, 3D printing, etc., are shaping the healthcare system [5]. Digitizing healthcare facilities and the provision of services to patients present numerous opportunities for enhancing patient outcomes, tracking data over time, and reducing human errors. Several medical specialists are benefiting from the emerging advances in AI [5]. For instance, in medical physics and radiation ecology, healthcare providers are using AI to aid in auto-segmentation prognostic prediction and decision-making. In radiology, facilities can screen, stage, and restage cancer, hence supporting structured reporting, incidental findings, and detection of imaging protocol optimization [6]. Although they are efficient in healthcare, they are fragile, hence offering inappropriate answers. This is especially true when confronted with images that are outside the healthcare providers' knowledge set.
A broader range of medical diagnoses in the healthcare sector is based on analyzing diseases through high digital services. For instance, the application of AI in the assessment of medical images has resulted in the accuracy of the evaluation of the images as it is performed automatically [5, 6]. Determining the impact of artificial intelligence on healthcare is very critical. In healthcare, AI is widely used on various grounds for better functioning of healthcare facilities to improve patients' healthcare outcomes and also ensure that patients can access services at a lower cost [5]. Saudi Arabia has highly adopted artificial intelligence as the country aims to improve the quality of services being offered to patients and promote easy access to healthcare services.
Even though most of the technological innovations used in KSA employ AI to interpret medical images, such as CT scans and MRI scans, the context of its use is very important. Saudi Arabia's healthcare sector presents unique challenges as well as opportunities for AI due to various factors, such as the effect of cultural and economic factors on the use and effectiveness of AI, which remains largely unexplored [7].
In the research study performed by Davenport and Kalakota [8], it was suggested that AI can perform better than humans in diagnosing diseases. The algorithm indicated AI to outperform human diagnosis. In KSA, most of the studies undertaken have focused on specific diagnoses. It should also be noted that KSA has a unique and diverse population compared to other developed nations where the effect of AI on diagnosis has been assessed, such as the study carried out by Tsang et al. [9] in Europe and the United States and the study by Soellner and Koenigstorfer [10] in Germany. In these advanced nations, AI has significantly enhanced the precision of diagnostic imaging, such as in the early detection of cancers and cardiovascular diseases. In contrast, many poor and underdeveloped nations face significant challenges in fully implementing AI technologies due to inadequate infrastructure, limited access to trained personnel, and financial constraints. The review by Zuhair et al. [11] showed that many developing nations in Latin America, Africa, and Asia lack stable economic growth, modern technologies, and research opportunities, and hence have limited applications of AI in health diagnosis. For example, the study by Onu et al. [12], conducted in Nigeria, showed that the effectiveness of diabetic retinopathy (DR) screening is often hindered by a lack of adequate eye care infrastructure. However, there has been much progress in the use of AI for DR screening both in Nigeria and Zambia, which shows great potential for specific and accurate diagnosis [12, 13]. This has also been evidenced in India where AI techniques have been used in examining chest X-rays, which has allowed for precise and prompt identification of tuberculosis [14]. Based on this, this research work sought to offer important insights to help the KSA healthcare policymakers and practitioners and also other regions in the Middle East.
The paper systematically evaluated the impact of the application of artificial intelligence on diagnosis accuracy in healthcare facilities. The systematic review sought to identify the artificial intelligence applications that have been evaluated for improving diagnostic accuracy in Saudi Arabia's healthcare, determine the diagnostic accuracy of these applications in KSA healthcare, and identify the challenges and opportunities of using artificial intelligence to improve diagnostic accuracy within Saudi Arabia’s healthcare.
2. METHODS
2.1. Search Strategy
The study employed a structured and systematic search strategy in compliance with the Preferred Reporting Items for Systematic Reviews and Meta-Analyses (PRISMA) criteria (Fig. 1) [15]. In this case, the researcher adopted an integrated search strategy to cover an extensive automated search of various online databases, complementing a manual review of the selected articles. An automated search strategy ensures that the most fitting online sources are integrated into the study [16]. Three databases were used to search the articles, including PubMed, Embase, and CINAHL, whereby appropriate filtering tools were used in each database to restrict the search results in order to minimize duplication [17]. The search for relevant articles linked to the impact of AI applications on diagnostic accuracy in the KSA healthcare sector was narrowed down to articles published between 2013 and 2023, as shown in the PRISMA diagram. The investigation covered several keywords to obtain the articles, including “artificial intelligence”, “AI”, “diagnostic imaging”, “diagnosis accuracy”, “diagnosis”, “machine learning”, “Saudi Arabia”, and “deep learning”. Also, it should be noted that AI is generally a broad-encompassing term and, hence, includes various AI techniques, such as neural networks, NLP, vector machines, and decision trees.
For the manual review, the researcher performed a broad review of the articles, which entailed reading the title of the study and the abstract and then skimming through the full content of the chosen articles to include relevant studies and exclude irrelevant studies [18]. Overall, the search of the articles was limited to studies being in English and published within the last 10 years.
2.2. Eligibility Criteria
To ensure that the systematic review produced accurate, objective, and meaningful results and that all literature conceived was relevant to the study, an eligibility criterion was put in place, as shown in Table 1.
Randomized controlled trials, quasi-experimental studies, or observational studies evaluating the impact of an AI application on diagnostic accuracy in a healthcare setting in Saudi Arabia and providing decision support to healthcare providers were included. However, the use of medical hardware devices, such as ultrasound machines, surgery robots, and X-ray machines, was outside the scope of the study. If well-established and executed, the eligibility criteria help reduce any risk of bias or errors and ensure that relevant studies are included in the review, hence leading to consistent conclusions.
2.3. Data Extraction and Charting
A data extraction form was developed and divided into three main parts: a) study characteristics relating to the author, year, study design, and participants; b) AI application characteristics, including application description, AI techniques, clinical tasks, and targeted diseases; c) study outcomes and key findings, including the performance of the AI applications, clinician outcomes, and patient outcomes. The form facilitated the analysis.
2.4. Data Analysis
The data from the retrieved articles were analyzed using the narrative approach. This approach is a qualitative method of data analysis that involves identifying and interpreting all the key findings of the included research studies. To meet the objective of the study, the following research questions were considered:
- What artificial intelligence applications have been evaluated for diagnostic accuracy in Saudi Arabia's healthcare?
- What is the diagnostic accuracy of these applications in KSA healthcare?
- What are the challenges and opportunities for using artificial intelligence to improve diagnostic accuracy in Saudi Arabia’s healthcare?
3. RESULTS
The initial search obtained a total of 450 articles from PubMed, Embase, and CINAHL databases. These articles were further evaluated based on the inclusion criteria of the study, whereby 400 duplicate records were excluded from the study. After that, 30 articles were excluded after assessing the articles based on the titles and the abstract content. Hence, 20 articles remained, and the researchers conducted a full-text assessment for eligibility, of which 8 articles were excluded and 12 articles were included for the review, as shown in Fig. (1).
3.1. Study Characteristics
Table 2 presents the characteristics of the reviewed studies, including the authors and year of publication, study design and the participants, and involved hospitals or countries. The analysis showed most of the studies, i.e., 11 out of 12, to be conducted between 2020 and 2023. It also showed that the last three years have witnessed the largest number of studies on artificial intelligence, which is probably because it is during this period that AI has gained much interest in the health sector. The included studies were found to be conducted in KSA and within different hospitals.
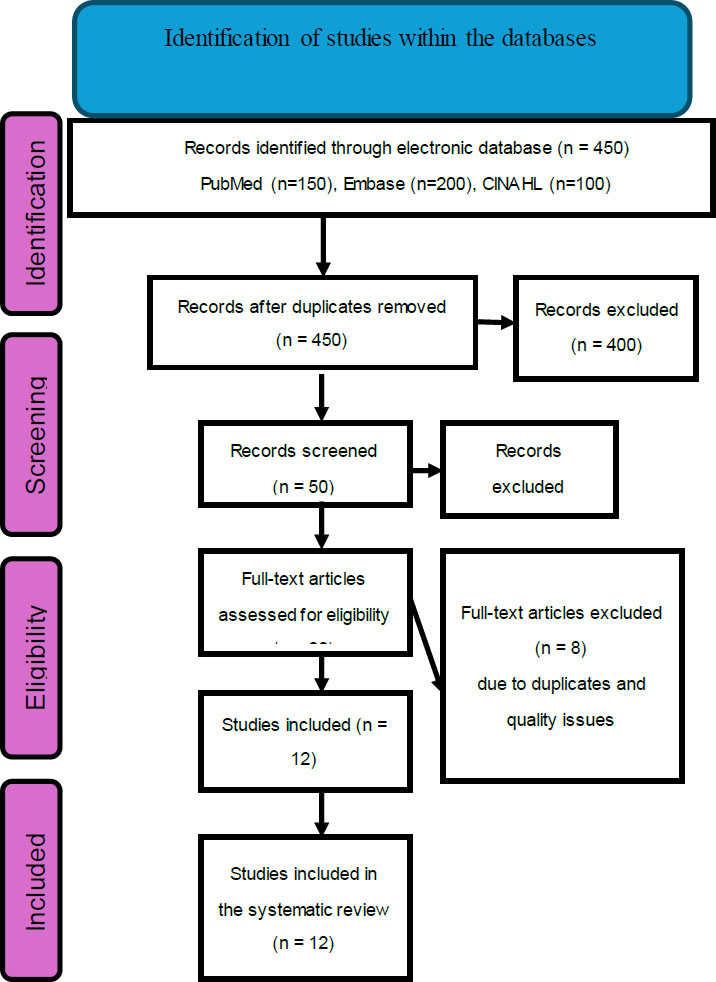
PRISMA flow diagram based on PRISMA guidelines.
Criteria | Inclusion | Exclusion | Rationale |
---|---|---|---|
Publication type | Scholarly articles | Reports, newsletters, conference abstracts, commentaries, reviews, and other sources | This made sure the retrieval of only information and data at the academic level. |
Peer-reviewed | Peer-reviewed | Non-peer reviewed | This ensured the high quality of articles used for the review. |
Year of publication | Only articles published between 2013 and 2023 | Articles published before 2013 | This ensured the validity of the content. AI technology is highly dynamic and 10 years is an appropriate period to give the researcher a good overview of the recent AI trends in healthcare. |
Language | English language | Arabic or any other language | English was the official language of all the articles included. |
Author and Year | Study Design | Sample | Hospital/Country | Findings and Uutcomes |
---|---|---|---|---|
Barakat, A. A. R. (2022) [20] | A cross-sectional study | 309 ophthalmologists and physicians involved in diabetic eye care |
Saudi Arabia | 79% of the respondents expected clinical efficiency to increase with the use of AI. 63% believed that it will decrease the workforce of physicians. |
Aldhafeeri, F. M. (2022) [37] | An online cross-sectional survey | 562 Saudi radiographers | Saudi Arabia | 88.5% stated that AI would improve the accuracy of diagnosis. Challenges reported included the high cost of equipment, inadequate knowledge, fear of losing employment, and concerns related to potential medical errors and cyber threats. |
Alghamdi, S. A. and Alashban, Y. (2023) [21] | A cross-sectional study | 129 radiologists | Saudi Arabia | The majority of the participants had a positive outlook. 17.0% of participants indicated concern that AI will replace their jobs. |
Khafaji et al. (2022) [7] | An observational cross-sectional study | 154 radiology residents | Saudi Arabia | 53% stated that AI will increase accuracy and reduce reporting workload, while 28% expected an increase in workload. 42% anticipated that job positions would decrease. |
Senan et al. (2021) [23] | An experimental study | 400 patients | Saudi Arabia | Artificial intelligence techniques were reported of great importance in the early detection of chronic kidney disease (CKD) |
Syed, W. and Basil A. Al-Rawi, M. (2023) [24] | A cross-sectional, questionnaire-based study | 157 senior pharmacy students | College of Pharmacy, King Saud University |
More than half of the participants believed that AI is facilitating healthcare professionals. 75.1% agreed that AI reduces errors in medical practice. |
Alelyani et al. (2021) [22] | A cross-sectional, questionnaire-based study | 714 radiology professionals | Saudi Arabia | 65% felt that the use of AI has made medical services more accurate. 89% of the participants thought that it would never replace radiologists. |
Mirza et al. (2022) | A cross-sectional study | 98 radiology trainees | Saudi Arabia | There was a positive perceived effect of AI on radiology workflow and the profession. It was expected to improve the accuracy of diagnosis and treatment in the field of medical imaging. |
Al-Hussain et al. (2022) [25] | An RCT | 1409 patients with voice disorders | Saudi Arabia | The most common technique used was the least square support vector machine with the highest accuracy. Quadratic discriminant analysis achieved the lowest accuracy and the K-nearest neighbor algorithm exhibited the highest sensitivity. |
AlAkeely et al. (2021) [27] | An observational study | Diabetic retinopathy (DR) patients | King Khaled Eye Specialist Hospital, Saudi Arabia | The automated fundus detector achieved an accuracy and recall of 99.20%. The classifier achieved an accuracy of 68.19% and a recall of 39.54%. |
Taylan et al. (2023) [26] | A retrospective observational study | 159 patients from family medicine and cardiology clinics at a university hospital in Saudi Arabia | Saudi Arabia | ML approaches, such as support vector regression (SVR), multivariate adaptive regression splines, the M5Tree model, and neural networks, were highly accurate in the detection of cardiovascular diseases (CVDs), showing their versatility and utility. |
BinDhim et al. (2015) [28] | A cross-sectional study | Participants aged 18 years and older | 66 countries, including Saudi Arabia | Smartphone apps were used as screening tools for depression and they exhibited increased accuracy. |
With regards to the study design, the 12 studies included 7 cross-sectional studies, 3 observational studies (1 retrospective study), 1 experimental study, and 1 randomized controlled trial (RCT). All the studies mentioned the participants or the sample characteristics ranging from healthcare professionals, involved hospitals or clinics, and patients.
3.2. Quality Assessment
The study quality assessment was done using the prediction model risk of bias assessment tool (PROBAST), given that the main aim was to estimate the quality of the prediction accuracy. This assessment was based on four main domains, including the participant domain, predictor domain, outcome domain, and analysis domain, as shown in Table 3. All 12 studies had a clearly defined study population, showing that the results can be generalized in KSA. Overall, the analysis showed the quality of the reviewed studies to be mixed, whereby some studies may have a higher risk of bias compared to others. From the analysis, 10 studies had a population that was representative of the target population, 8 studies were adequately controlled, and 9 of the studies reported model performance more transparently. Overall, the PROBAST analysis suggested the overall evidence based on AI's role in diagnostic accuracy in KSA to be relatively weak, but some of the studies [19-27] were promising, as they showed a lower risk of bias. On the other hand, BinDhim's study [28] did not meet the criteria for the predictor’s outcome domain and analysis as it was majorly a feasibility study. However, with respect to the overall rating of the four domains, a review of all the articles showed a relatively high rating, implying the evidence provided in the articles reviewed to be strong that AI can be used to improve diagnostic accuracy in KSA healthcare.
Domain | Rating | Explanation |
---|---|---|
Participant domain | High | All the studies included in the review involved a representative sample of patients from the target population, mainly including professionals, such as radiologists, radiographers, ophthalmologists, and physicians. |
Predictor domain | High | The AI applications were well-defined and reproducible. |
Outcome domain | High | The studies measured clinically relevant outcomes using standardized methods. |
Analysis domain | High | The studies used appropriate statistical methods to analyze the data. |
3.3. Artificial Intelligence Methods used in Studies and their Diagnostic Accuracy
The analysis of the studies showed the use of several artificial intelligence as well as machine learning methods in different studies and it was evaluated as to how they affect diagnostic accuracy in KSA. Taylan [26] studied support vector regression (SVR), multivariate adaptive regression splines, the M5Tree model, and neural networks. The least square support vector machine, quadratic discriminant analysis, and K-nearest neighbor algorithm were studied by Al-Hussain [25]. The deep learning technique was used by Barakat, and logistic regression and ROC analysis were employed by Senan [23] and Taylan [26]. Overall, these studies considered different sets of features or predictors, including the demographics of the patients, their disease history and body measurements, medications, procedures, and health service use.
Most of the studies applied AI methods either for prediction purposes or diagnostic purposes, and many of the studies did not compare different AI techniques, except for a few, such as AlAkeely et al. [27] showed that automated fundus detector achieved accuracy and recall of 99.20% and that the classifier achieved an accuracy of 68.19% and a recall of 39.54%, respectively. While Al-Hussain [25] stated the most common technique to be the least square support vector machine with the highest accuracy. Quadratic discriminant analysis achieved the lowest accuracy and the K-nearest neighbor algorithm demonstrated the highest sensitivity. Overall, most of the studies showed AI models and ML techniques to achieve high diagnostic accuracy of more than 50%. Studies by Senan [23], Barakat [20], Al-Hussain [25], and AlAkeely [27] showed most of the techniques to have high diagnostic accuracy of more than 90%, suggesting AI to have great potential for higher diagnostic accuracy in medical conditions, such as chronic kidney disease, cancer, and diabetes.
4. DISCUSSION
This section of the study discusses the results based on the reviewed literature. The discussion is provided to answer the research questions guiding this study. It concludes by providing the study's implications.
4.1. What Artificial Intelligence Applications have been Evaluated for Diagnostic Accuracy in Saudi Arabia’s Healthcare?
The reviewed research studies conducted in KSA in the last 10 years showed a wide range of AI applications to have been used and evaluated for diagnostic accuracy in healthcare in Saudi Arabia, including medical and diagnostic imaging analysis tools [19-21]; AI-based systems to detect and diagnose diabetes, chronic kidney disease, and other metabolic disorders [6, 27, 20, 22]; AI-powered voice analysis tools [24]; AI-based systems for early prediction and classification of diseases [25]; AI-driven mental health apps and chatbots [22]; and AI-based systems for the detection and classification of diseases, such as cardiovascular diseases (CVDs), for e.g., natural language processing (NLP) [25].
These results have shown the health sector in KSA to use a wide range of AI applications, whereby most of the AI applications have been found to be employed in areas, such as radiology for chronic kidney disease and diabetes, and medical imaging and prediction of diseases, such as cardiovascular diseases (CVDs). The AI uses machine learning models to simulate the human intellect and is usually based on statistical analysis of past data. As noted by Zanella-Calzada et al. [3], with the introduction of AI in healthcare, major fundamental shifts have been witnessed in healthcare through the application of automatic diagnosis, predictive measurements, and diagnostic tools [27]. The analysis also showed that all aspects of modern AI technology have been applied in KSA healthcare for diagnosis, replacing the more traditional methods. Therefore, the analysis has shown the AI applications in KSA to be employed in medical imaging analysis, such as radiology, used to analyze X-rays, CT scans, and MRIs, for disease diagnosis and risk prediction, such as in heart disease and diabetes, and even to assess the mental wellbeing [29].
4.2. What is the Diagnostic Accuracy of these Applications in KSA Healthcare?
Being able to make earlier diagnoses of diseases is one of the most important aims of modern healthcare if they are to be managed well and without much cost. As noted by Chang et al. [30], early detection of any disease, especially chronic diseases, reduces the burden of the disease and the need for other complex treatment procedures that enhance the outcome of the treatment. The review of the studies showed that even though many of the AI applications in KSA are new developments, they have demonstrated great performance in terms of their diagnostic accuracy, although this might vary depending on factors, such as clinical experience [31]. The findings have shown most of the AI applications to achieve an accuracy of more than 90%. The study by AlAkeely et al. [27] showed that an automated fundus detector achieved an accuracy and recall of 99.20%, and Senan et al. [23] found that an AI-based system was able to diagnose chronic kidney disease with an accuracy of 98.5%. This was found to be significantly higher than the accuracy of human experts, who could achieve accuracy rates of around 70-80% in chronic kidney disease diagnosis. Another study by Taylan et al. [26] found that an AI-based system was able to predict the risk of developing cardiovascular disease with an accuracy of 85%. This was significantly higher than the accuracy of human experts, who could achieve accuracy rates of around 60-70% for cardiovascular disease risk prediction [32-36]. Aldhafeeri showed that an AI-powered system for breast cancer detection achieved a diagnostic accuracy of 95%, and Al-Hussain et al. showed an accuracy of 92%. All these results showed promising diagnostic accuracy for the use of AI in KSA. These findings have been found to be consistent with the results of studies in other parts of the world. For example, some studies [28], [30] have shown AI technologies in areas, such as dentistry, to exhibit great performance in tooth detection, and diagnosing and predicting oral cancer and tumors. The study by Zanella-Calzada et al. [3] showed that most of the AI models achieved an accuracy of more than 0.69 in classifying individuals based on the type of disease. Overall, all these studies showed that AI is a very promising technology when it comes to enhancing diagnostic accuracy in the healthcare sector. However, it is important to consider different factors, such as their specific application, the hospital settings, the clinicians' experience, and the dataset quality [33-36].
4.3. What are the Challenges and Opportunities of using Artificial Intelligence to Improve Diagnostic Accuracy in Saudi Arabia’s Healthcare?
Overall, despite the promising results of the AI applications in KSA, the analysis also showed that some challenges need to be addressed if the technology is to achieve the intended objective. The studies by Barakat [20], Aldhafeeri [37], Alghamdi and Alashban [21], and Khafaji et al. [7] showed that many physicians fear their jobs from being replaced by AI applications. Barakat [20] noted that 63% of the participants believed that AI would decrease the workforce of the physicians, and Alghamdi and Alashban [21] indicated that 17.0% of participants indicated concerns that AI will replace their jobs. Khafaji et al. [7] stated that 42% of the participants anticipated that job positions would decrease and 28% expected an increase in workload. These findings have been found to align with the findings of other researchers, such as Tursunbayeva and Renkema [2], who have noted that the jobs of many people might be at risk due to changes in job design and job demands, while Federspiel et al. [32] stated that while AI offers important solutions in healthcare, it might render human labor increasingly obsolescent.
Other challenges in KSA due to AI, as listed by Aldhafeeri [37], include high cost of equipment, inadequate knowledge, fear of losing employment, and concerns related to potential medical errors and cyber threats. This aligns with the study by Zuhair et al. [11], which showed that many developing nations’ adoption of AI is hindered by factors, such as high installation and maintenance costs of equipment and connectivity issues. These barriers lead to limited AI adoption, resulting in outdated diagnostic methods that lead to lower-quality patient care. In contrast, as seen in this review, Saudi Arabia, although still developing its AI healthcare infrastructure, has made significant strides by investing in healthcare innovation, including AI research and technology integration [19-21]. Hence, despite these challenges, the analysis showed that there are several opportunities for using AI to enhance diagnostic accuracy in KSA. As the nation seeks to enhance and improve the healthcare sector in line with Vision 2030, AI can help address the shortage of healthcare professionals and improve the quality-of-care outcomes by offering decision support [38-43] and improving the accessibility of healthcare professionals, thus making it easier to offer accurate and timely diagnoses to patients in remote areas. All these data have also been found to align with studies conducted in African nations, such as Nigeria and Zambia, which have shown artificial intelligence to present more cost-efficient alternatives to traditional treatment methods, especially in rural areas [12, 13]. This underscores the importance of continued efforts to bridge these gaps by ensuring that AI's diagnostic capabilities reach underserved populations and improving the overall quality of care across the country.
CONCLUSION
From the analysis, it has become evident that artificial intelligence models have been widely explored for the diagnosis of different diseases globally and many of the studies have shown that the models can achieve higher accuracy levels. In KSA, the analysis has shown the increasing use of AI in healthcare, enhancing the overall healthcare outcomes and being helpful in a wide variety of diseases and conditions, including chronic diseases. The models have shown the use of AI as capable of enhancing diagnostics and treatment quality, which can be essential in planning for preventing care in line with Vision 2030. Even though there have been some challenges indicated, such as fear of the loss of jobs and issues related to security and costs, KSA is on the right pathway as the government invests in technology to improve healthcare, which has great potential to significantly improve the diagnostic accuracy of various diseases.