All published articles of this journal are available on ScienceDirect.
Association between Continuity of Primary Care and Chronic Conditions among Patients in Guangdong Province, China
Abstract
Background:
One important aspect of primary care is the association between continuity with primary care providers (PCPs) and chronic conditions among patients. However, there is a lack of literature to specifically address these issues in countries with large populations, such as China.
Objective:
The goal of this study is to examine the effect of chronic conditions on the continuity of primary care among patients from a cross-sectional survey conducted in Guangdong province, China.
Methods:
Multiple logistic regression was used to estimate the effect of chronic conditions on continuity. Effect modification by residency status (migrants vs. locals) on the relationship between the number of chronic conditions and continuity was also assessed. The intermediate effect of the existing contract between patients and PCPs was also evaluated for examining the relationship between chronic conditions and continuity of primary care.
Results:
Participants with chronic conditions had 2.32 (95% CI: 1.78 - 3.04) times the odds to continue with their PCPs for one year or more. Compared to those without any chronic condition, individuals with one chronic condition had 2.03 (95% CI: 1.49 - 2.75) times, and more than one chronic condition had 3.00 (95% CI: 2.01 - 4.49) times the odds to continue with their PCPs. The residency status of the participants did not modify the effect of the number of chronic conditions on the continuity of primary care. The contract between patients and their PCPs was found to be an intermediate factor for the relationship between chronic conditions and continuity.
Conclusion:
To strengthen the connection between patients with chronic conditions, PCPs may reinforce the relationship between providers and patients, thus ultimately benefitting the patients. The results of this study can serve as a reference for policymakers.
1. INTRODUCTION
Chronic conditions kill 41 million people each year, which accounts for 74% of all deaths globally and a majority of deaths are from developing countries [1]. The surge in chronic conditions may lead to increases in disease burden, overwhelmed healthcare facilities, and strained productivity [2, 3]. The UN has targeted to reduce the deaths from chronic conditions to one-third from 2015 to 2030 [2, 3]. Access to primary care, early detection, preventive measures, and treatment is crucial to achieving this target [4]. Among these measures, access to primary care could be the most cost-effective way to reduce deaths.
Primary care incorporates six core functions: accessibility, first contact, continuity, comprehensiveness, coordination, and patient-centered care [5, 6]. All these elements are coequally essential for the intervention against all chronic diseases. However, among these six core functions, continuity is important to improve the prevention of chronic illnesses, ensure early detection [7], reduce hospitalizations and readmissions [8, 9], and efficiently manage patients’ health information [10]. Compared to a new primary care practitioner, a family clinician can effectively diagnose the patients' symptoms based on the most up-to-date, relevant patient history and experience in evaluating patient-specific needs. All these play an important role in minimizing diagnosis to treatment time [10]. The continuity of primary care is defined as the continuation (frequency of the visits being repeated over time) and coordinated care (consistency of treatment plan with appropriately organized information) along with the cooperative relationship between the patients and their providers [11]. The emphasis of continuity is to lessen the healthcare system burden, specifically, to reduce readmission, stop exhausting hospital resources, and reduce traumatic experiences and expensive hospitalization [3, 9, 12, 13]. The issue related to the continuity of primary care has drawn the attention of many researchers worldwide. For example, some researchers found an association between continuity of care and emergency department use. Other investigators reported that higher continuity of care substantially reduced the use of emergency health care for the elderly [14]. Continuity of primary care is reported to be helpful with the early detection of chronic conditions, such as diabetes [15], asthma [16], hypertension [17], and cancer [7, 18] in many studies. For example, early diagnosis increases the survival of cancer patients. Women who have a long-term patient-provider relationship with the same medical clinician were more likely to undergo screening for different types of cancer, such as breast or cervical cancer. An evidence-based study conducted mostly in Canada and Taiwan area reported that higher continuity of care led to a lower rate of hospitalization and was associated with escalated satisfaction among patients suffering from chronic illnesses [19].
China is the largest developing country in terms of population. According to World Health Organization (WHO) report published in 2015, the proportion of people aged higher than 60 years would double in the next 20 years in China [20]. As a result, the disease burden in China would lean toward chronic diseases. Chronic diseases, such as ischemic heart disease, stroke, chronic obstructive pulmonary disease (COPD), and type 2 diabetes, account for 80% of death of people aged higher than 60 years [21]. To resolve this complex situation and divert the increasing pressure on the healthcare system, the Government of China has decided to adopt a target of restructuring the country's healthcare system by establishing stronger primary healthcare services [22]. Even though a significant amount of financial investment and accommodative policies to promote primary care have been adopted, many challenges still prevail [23]. Among these challenges and barriers, lack of educational and occupational training for the general practitioner [24, 25], insufficient coordination between different levels of the healthcare system, and a gap in the continuity of care are top-ranked issues [23]. Hence, taking necessary actions to improve the continuity of primary care facilities is a must. However, there is a lack of literature to specifically address this problem in countries with huge populations, such as China. In this research, we investigate the relationship between the continuity of primary care and the pattern of chronic conditions in Guangdong, China, which would potentially provide evidence for health professionals, researchers, and policymakers to improve the healthcare system.
To our knowledge, this is the first study done in China that incorporates quantitative measurements on continuity of care using a specific question from our survey as the proxy for encounter-level data from electronic health records (EHRs). The survey questions included information regarding the length of the relationship with the primary care physician, which facilitated categorizing the continuity into 1-year and 3-year time intervals (k1, k3), which usually could only be calculated using EHRs. Additionally, we asked the participants about their number of chronic conditions to evaluate the association between the number of chronic conditions and continuity, which was not reported in previous works, such as the recent study by Zhang et al. [26]. The primary goal of this research is to investigate the effect of the number of chronic conditions on continuity based on our survey. Our results can add to the current knowledge regarding primary care services. In addition, we evaluate whether the relationship between chronic conditions and continuity is different across different residency statuses.
The rest of the paper is organized as follows; Section 2 describes the data collection approach and analytical methods, including the study design and corresponding measures of the analysis. Section 3 reports the results of the analysis. Section 4 discusses the results, summarizes our key findings, and reports the study's limitations. Finally, section 5 presents the conclusions.
2. MATERIALS AND METHODS
2.1. Study Design
The data used in this study was extracted from a cross-sectional survey that was conducted in Guangdong province located in southern China in 2019 between January and March. The samples were obtained through a three-stage cluster sampling technique. The first stage of the process constituted a sampling of 10 cities. Probability proportional to size (PPS) without replacement was conducted based on the structure of the population and the availability of primary care resources. Among those cities, 16 healthcare-providing organizations were selected using the PPS sampling method in the second stage. Finally, the participants were invited to take part in the survey willingly in the waiting area of the healthcare organizations. The Institutional Review Board (IRB) of Sun Yat-sen University reviewed and approved the protocol of the study in compliance with the Declaration of Helsinki (Medical Ethics (2018) 014) [27]. More details about the data collection and sampling procedure could be found in the study by Liao et al., which was also based on the same study [28]. To ensure the accuracy of the data, the overall process of the interview was carried out by five postgraduate students majoring in public health who received advanced training from two researchers. The criteria for a patient to be eligible to participate in the survey were; the participants were at least 18 years of age or older, the participants visited the same community health center three or more times, the participants were able to communicate properly whether in Mandarin or Cantonese, who gave verbal consent to participate in the study voluntarily, and did not have any history of mental illness or had been poor physical health condition or were unable to comprehend the interview questions. The purpose of the study and the consent form were explained to each participant during the interviews with clarity, and verbal consent was ensured before conducting the interview. A small incentive worth about 1 USD was given as a token of appreciation for the contribution. Participants were given the independence to opt out of the survey at any time. Strict confidentiality was maintained in collecting, handling, and managing the data by the supervisor and data administrator to account for any sort of information biases. An intensive interview process was carried out to make sure there was an ignorable number of missing values.
2.2. Measures
The measures used in this study were all extracted from the survey questionnaire. A brief explanation of the measurements is given below:
2.2.1. Outcomes
The primary outcome variable was continuity, measured in three ways based on the Assessment Survey of Primary Care (ASPC). This scale was newly developed by Kuang’s research group and aimed to evaluate patient-perceived primary care quality [29]. Continuity was one of the six dimensions of APSC. It considered the visiting times, the knowledge of the patients/family, and the active contact with patients, making the measurement of relationship continuity more comprehensive [29]. Our first two outcomes were based on the item about the length of the relationship between the patients and the provider (1 = less than 1 year; 2 = 1-3 years; 3 = 3-5 years; 4 = More than 5 years). Firstly, we re-categorized this variable into two groups, less than one year and equal to or greater than one year. It represented the continuity of less/more than one year to create the variable for measuring continuity of at least one year or more (k1). Secondly, we categorized this variable again into two groups, less than three years and equal to or greater than three years, which represented the continuity of care less/ more than three years to create the variable for measuring continuity of care for at least 3 years or more (k3).
Our third outcome was the average domain score of the seven items in the continuity dimension, which is shown in supplementary materials. The domain score was further standardized to a percentage score on a 0-100 scale. Specifically,
Average domain score = The sum of items under continuity/the number of items.
Standardized (percentage) score = (The sum of items under continuity /the number of items) *25.
2.2.2. Exposures (Chronic Conditions)
In the interview, participants were inquired about their health condition. They were asked if they were diagnosed with a chronic illness(s) (Yes/No) and the number of chronic diseases they were diagnosed with (17 options accounted for 17 chronic diseases). The number of chronic diseases experienced was divided into three categories (no chronic illness/ single chronic illness present/ multiple chronic illnesses present). In this study, both “chronic illness (Yes/No)” and “number of chronic illnesses” were used as exposures.
2.2.3. Covariates
The survey questionnaire included other socio-demographic information about the study participants. Age (between age 18 to 60/ more than 60), gender, residence status (migrant, local), insurance status (yes, or no), household income per capita per month (less than 5000/ between 5000-10,000/ more than 10,000 per month), employment situation (no employment, some employment, and full employment), and education level (primary school or below/ secondary or high school/ bachelors or higher degree) were included as covariates. The socio-economic status score (SES) was created by combining education level, household income per capita per month, and income status and divided into three categories (low, medium, and high). Some participants reported having a contract with the primary care practitioner, which might act as a mediator on the effect of chronic illness conditions on continuity of care. Contract services (have a contract with the PCP/ do not have a contract with the PCP) were used to explore the mediation effect. Fig. (1) shows the directed acyclic graph for the association between chronic conditions and continuity with PCPs.
2.3. Statistical Analysis
Data were summarized as mean ± standard deviation (SD) for continuous variables satisfying the normality assumption and as frequency (N) and percentage (%) for categorical variables. One of the goals of this study was to investigate the key factors affecting the continuity of primary care. To achieve this goal, a multiple logistic regression model [30] was used to explore the covariates selected based on the literature review and estimate the effect of chronic conditions on continuity. Additionally, the interaction term of a migrant with chronic conditions was included in the logistic regression model to examine whether the residency status of being a local or a migrant modifies the effect of chronic conditions on the continuity of primary care. Chi-squared (χ2) and asymptotic proportion tests were carried out for the comparison of attributes between different subgroups of individuals, for example, individuals with and without chronic conditions.
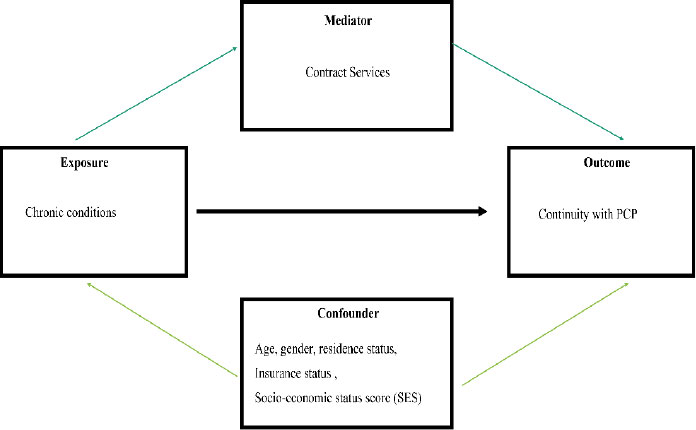
To explore the association between chronic conditions and continuity of primary care, three exposure variables representing chronic conditions were considered. Firstly, the variable chronic binary, which was dichotomized into two categories representing the absence and presence of the chronic conditions; secondly, chronic trichotomized, which has three categories representing the presence of none, one and more than one chronic illnesses, and finally, domain score, which was a continues variable representing the score of the continuity domain on a scale of 100. Logistic and linear regression models used for the analysis are shown below;
For categorical outcome variable,
![]() |
(1) |
Here, Y represents the outcome variable, X1 represents the primary exposure, and X2 represents the residency status, and X3, . . .,Xk represents the rest of the confounding variables. Here, β1,β2,β3, . . .,βk are the coefficients explaining the corresponding effect of the variables included in the model. Furthermore, after including the interaction, we obtained:
![]() |
(2) |
Where γ refers to the interaction effect between exposure and effect modifier.
For continuous outcome variables:
![]() |
(3) |
Here, Y represents the outcome variable, X1 represents the primary exposure and X2 represents the residency status and X3, . . .,Xk represents the rest of the confounding variables. Here β1,β2,β3, . . .,βk are the coefficients explaining the corresponding variables.
![]() |
(4) |
Where γ refers to the interaction effect between exposure and effect modifier.
All tests conducted in the above analyses were two-sided, and p-values < 0.05 indicates statistically significant results. Statistical analytical software Stata (Stata Corp., College Station, TX, USA) MP version 16 and R (cran.r-project.org) was used for all statistical analyses.
Table 1.
- | - |
Individuals without CC* (N = 649) |
Individuals with CC* (N= 536) |
Total (N = 1185) |
Chi-square (χ2) p-value** |
Gender | Male | 217(48.12%) | 234(51.88%) | 451(38.06%) | <0.001 |
Female | 432(58.86%) | 302(41.14%) | 734(61.94%) | ||
Age | 18 – 60 | 584(70.26%) | 232(29.74%) | 780(65.82%) | <0.001 |
> 60 years | 101(24.94%) | 304(75.06%) | 405(34.18%) | ||
Residency Status | No | 305(44.72%) | 377(55.28%) | 682(57.55%) | <0.001 |
Yes | 344(68.39%) | 159(31.61%) | 503(42.45%) | ||
Medical Insurance | No | 93 (71.54%) | 37(28.46%) | 130(10.97%) | <0.001 |
Yes | 556(52.70%) | 499(47.30%) | 1055(89.03%) | ||
SES Score | Low | 251(42.47%) | 340(57.53%) | 591(49.87%) | <0.001 |
Median | 299(64.72%) | 163(35.28%) | 462(38.99%) | ||
High | 99 (75%) | 33(25%) | 132(11.14%) | ||
Education | Primary school or below | 129 (41.08%) | 185(58.92%) | 314(26.50%) | <0.001 |
Secondary/ High School | 446 (58.68%) | 314(41.32%) | 760(64.14%) | ||
Bachelor of Higher Degree | 74 (66.67%) | 37(33.33%) | 111(9.37%) | ||
Household Income (CNY/ month) | <= 5000 | 403 (49.51%) | 411(50.49%) | 814(68.69%) | <0.001 |
5000-10,000 | 114 (65.52%) | 60(34.48%) | 174(14.68%) | ||
>=10,000 | 132 (67.01%) | 65(32.99%) | 197(16.62%) | ||
Employment Status | No employment | 160 (54.79%) | 132(45.21%) | 292(24.64%) | <0.001 |
Some employment | 101 (27.98%) | 260(72.02%) | 361(30.46%) | ||
Fully employed | 388 (72.93%) | 144(27.07%) | 532(44.89%) |
** P- values are based on Chi-squared (χ2) test of differences between those with chronic conditions and those without chronic conditions.
3. RESULTS
3.1. Participants Characteristics
The total number of primary care patients included in the survey was 1,185, among which 217 (38.06%) were males and the rest were females (61.94%). Among these primary care patients, the number of individuals with chronic conditions was 536, accounting for 45.23% of the total participants. Table 1 reports the health and demographic characteristics of the respondents. Chi-square (χ2) tests were conducted to compare the health and demographic characteristics of respondents with and without chronic conditions.
More than half of the participants were local, and around 40% migrated from elsewhere. Almost 90% of the total patients had medical insurance. There was a significant difference in all characteristics between patients with chronic conditions and without such conditions. The proportion of males with chronic conditions (51.88%) was higher than that of females (41.14%). Similarly, chronic conditions were more common among the participants aged 60 years and above (75.06%) than the younger participants (29.74%). Due to an ignorable number of missing data, no sensitivity analysis was performed.
3.2. Effect of Chronic Illness Condition of the Patients in the Continuity of Care
To explore the effect of the chronic conditions of the participants on the continuity of care, an unadjusted model (Model 1), an adjusted model (Model 2), an unadjusted model with residency status as an effect modifier (Model 3), and an adjusted model with effect modifier were conducted for all the outcome variables (Model 4).
Table 2 illustrates the logistic regression results of these four models, where the outcome variable is binary (yes/no) continuity for one year or more (k1).
Results from the unadjusted logistic regression model indicated that, compared to the participants without any chronic conditions, participants with such conditions had 2.32 (95% CI: 1.78 – 3.04; p < 0.001) times the odds to continue with their PCPs for one year or more (Table 2). It was further observed that the number of chronic conditions from which participants were suffering was a significant factor. In comparison with those without chronic conditions, individuals with one chronic condition had 2.03 (OR = 2.03; 95% CI: 1.49-2.75; p <0.001) times the odds, and individuals with more than one chronic condition had 3.00 (OR = 3.00; 95% CI: 2.01- 4.49; p <0.001) times the odds to have continuity with the same PCP for more than a year compared to those who have no chronic conditions.
The adjusted logistic regression model considering confounders where chronic conditions (binary) were considered as the exposure (Table 3) also showed similar results with a significant effect of chronic conditions on continuity of care. However, the adjusted odds ratio decreased to 1.72 (95% CI: 1.27 – 2.32; p < 0.001). It was also noticed that the variable indicating the residency status of the participant in the adjusted model without interaction was found significant (OR = 0.42; 95% CI: 0.30 – 0.5; p < 0.001). The socio-economic score (SES) of the participants was also significant. The odds of continuing primary care with the same primary care for one year or more were higher for individuals with a median SES compared to those with a higher SES.
OR* | P | 95% CI** | Forest Plot on odds ratio | |
Model 1 | - | - | - | ![]() |
Chronic Condition Binary | - | - | - | |
No | Ref | Ref | Ref | |
Yes | 2.32 | <0.001 | 1.78,3.04 | |
Chronic Condition Categorical | - | - | - | |
No | Ref | Ref | Ref | |
One | 2.03 | <0.001 | 1.49,2.75 | |
More than one | 3.00 | <0.001 | 2.01,4.49 | |
Model 2 | - | - | - | |
Chronic Condition Binary | - | - | - | |
No | Ref | Rref | Ref | |
Yes | 1.73 | <0.001 | 1.27,2.34 | |
Chronic Condition Categorical | - | - | - | |
No | Ref | Ref | Ref | |
One | 1.72 | 0.001 | 1.23,2.39 | |
More than one | 1.75 | 0.017 | 1.11,2.78 | |
Model 3 | - | - | - | |
Chronic Condition Binary | - | - | - | |
No | Ref | Ref | Ref | |
Yes | 2.15 | <0.001 | 1.47,3.14 | |
Chronic Condition Categorical | - | - | - | |
No | Ref | - | - | |
One | 2.05 | 0.002 | 1.31,3.20 | |
More than one | 2.30 | 0.001 | 1.40,3.79 | |
Model 4 | - | - | - | |
Chronic Condition Binary | - | - | - | |
No | Ref | Ref | Ref | |
Yes | 1.77 | 0.007 | 1.17,2.69 | |
Chronic Condition Categorical | - | - | - | |
No | Ref | Ref | Ref | |
One | 1.74 | 1.74 | 1.09,1.80 | |
More than one | 1.82 | 1.82 | 1.05,3.12 |
Table 3 represents the linear regression results of these four models where the outcome variable was domain score, at first using binary chronic condition as the exposure and then using categorized chronic condition as the exposure.
Domain score is not only the measure of continuity but it can also be considered the quality of the patient-provider relationship. An increase in the domain would indicate better communication between the PCP and patients, leading to better care. Similar to the results from the logistic regression models, adjusted linear regression analysis using domain score as the outcome variable showed a significant effect of chronic conditions on continuity of care. Based on the unadjusted model where chronic conditions were considered as the exposure, the domain score of continuity with the same primary care practitioner was 8.19 (95% CI: 6.36 - 10.36; p < 0.001) units higher among the individuals with chronic conditions compared to the participants without them (Table 4). Furthermore, with an increase in the number of chronic conditions from one to more than one, the domain score increased twice compared to the individuals with no chronic conditions (Table 4).
Moreover, by focusing on the marginal effects of the estimated mean continuity domain score of the number of chronic conditions, it was found that the higher the number of chronic conditions, the higher the continuity (Fig. 2). Furthermore, To explore the effect of chronic conditions on the continuity categorized into 3 years intervals, an unadjusted model (Model 1), an adjusted model (Model 2), an unadjusted model with residency status as an effect modifier (Model 3), and an adjusted model with effect modifier were conducted (Model 4).
Table 4 illustrates the logistic regression results of these four models, where the outcome variable was continuity for three years or more (k3), first using binary chronic conditions (yes, no) as the exposure and then using categorized chronic conditions as the exposure.
There was no significant association found between chronic conditions binary and continuity of care with the same PCP for 3 years or more (Model 2) from the results of the adjusted model Table 4. It was also found that for the categorical outcome variable that considered multiple chronic conditions, there was no significant association with continuity as well. It indicated that in terms of long-term continuity with the same PCP, chronic conditions seemed to have no effect.
- | Coef.* | P | 95% CI** | Forest Plot on Coefficients |
Model 1 | - | - | - | ![]() |
Chronic Condition Binary | - | - | - | |
No | Ref | Ref | Ref | |
Yes | 8.19 | <0.001 | 6.36-10.35 | |
Chronic Condition Categorical | - | - | - | |
No | Ref | Ref | Ref | |
One | 5.99 | <0.001 | 3.92-8.07 | |
More than one | 12.30 | <0.001 | 9.71-14.89 | |
Model 2 | - | - | - | |
Chronic Condition Binary | - | - | - | |
No | Ref | Ref | Ref | |
Yes | 3.75 | <0.001 | 1.84-5.67 | |
Chronic Condition Categorical | - | - | - | |
No | Ref | Ref | Ref | |
One | 3.08 | 0.003 | 1.02-5.14 | |
More than one | 5.54 | <0.001 | 2.78-8.31 | |
Model 3 | - | - | - | |
Chronic Condition Binary | - | - | - | |
No | Ref | Ref | Ref | |
Yes | 6.71 | <0.001 | 4.39-9.04 | |
Chronic Condition Categorical | - | - | - | |
No | Ref | Ref | Ref | |
One | 5.19 | <0.001 | 2.53-7.85 | |
More than one | 8.84 | <0.001 | 5.89-11.78 | |
Model 4 | - | - | - | |
Chronic Condition Binary | - | - | - | |
No | Ref | Ref | Ref | |
Yes | 3.66 | 0.004 | 1.19-6.15 | |
Chronic Condition Categorical | - | - | - | |
No | Ref | Ref | Ref | |
One | 2.88 | 0.040 | 0.14-5.62 | |
More than one | 5.17 | 0.001 | 2.03-8.31 |
Table 4.
OR* | P | 95% CI** | Forest Plot of OR | |
Model 1 | - | - | - | ![]() |
Chronic Condition Binary | - | - | - | |
No | Ref | Ref | Ref | |
Yes | 1.53 | 0.001 | 1.18-1.99 | |
Chronic Condition Categorical | - | - | - | |
No | Ref | Ref | Ref | |
One | 1.28 | 0.106 | 0.94-1.74 | |
More than one | 2.04 | <0.001 | 1.44-2.89 | |
Model 2 | - | - | - | |
Chronic Condition Binary | - | - | - | |
No | Ref | Ref | Ref | |
Yes | 0.95 | 0.762 | 0.70-1.29 | |
Chronic Condition Categorical | - | - | - | |
No | Ref | Ref | Ref | |
One | 0.91 | 0.614 | 0.66-1.29 | |
More than one | 1.02 | 0.894 | 0.68-1.54 | |
Model 3 | - | - | - | |
Chronic Condition Binary | - | - | - | |
No | Ref | Ref | Ref | |
Yes | 1.54 | 0.009 | 1.11–2.12 | |
Chronic Condition Categorical | - | - | - | |
No | Ref | Ref | Ref | |
One | 1.40 | 0.074 | 0.97-2.04 | |
More than one | 1.72 | 0.008 | 1.15-2.56 | |
Model 4 | - | - | - | |
Chronic Condition Binary | - | - | - | |
No | Ref | Ref | Ref | |
Yes | 1.12 | 0.551 | 0.78-1.59 | |
Chronic Condition Categorical | - | - | - | |
No | Ref | Ref | Ref | |
One | 1.07 | 0.731 | 0.71-1.60 | |
More than one | 1.18 | 0.466 | 0.76-1.83 |
3.3. Potential Effect of Residency Status on the Association between Chronic Illnesses and Continuity of Care
Considering the residency status of the participants as a potential effect modifier, we conducted both logistic regression and linear regression. Table 5 depicts the results of the logistic regression and linear regression models, including effect modifiers (Equations 2 and 4) for both binary and categorized chronic conditions.
The results indicated no statistically significant interaction between residency status and chronic conditions, ruling out the possibility of being an effect modifier. This makes it evident that the association between continuity of care and chronic conditions was not affected by whether the participant is a local resident or migrated from a rural area.
4. DISCUSSION
Chronic conditions had a significant effect on the continuity in terms of dichotomized visit-based outcome (k1, k3) and the continuity domain score. Our results indicated the agreement between the visit-based measures (k1, k3) and the comprehensive continuity score. Besides, an increase in the number of chronic conditions significantly increased the chance of continuity of PCPs. Our results were consistent with previously published studies in China. A recent cross-sectional study conducted in Hangzhou, China reported that chronic conditions (yes vs. no) were a significant contributing factor to continuity in urban areas of China [26]. The study also found that continuity with their general practitioners (GPs) was normally overlooked by patients. A recent study on 700 participants from an urban area of Beijing assessed the relationship between continuity and patients’ preferences in their relationships with their PCPs. This study concluded that the relationship preferences of patients are associated with their experience of continuity of care, and patients’ strong preference for free choice of doctors is not aligned with relational continuity with primary care, a desirable feature of cost-effective healthcare systems [31]. The GPs in China can normally take care of patients with different types of chronic diseases, so patients with multiple chronic conditions may have weaker preferences for free choice of providers. This may partially explain the stronger continuity with PCPs/GPs among patients with more chronic conditions in China.
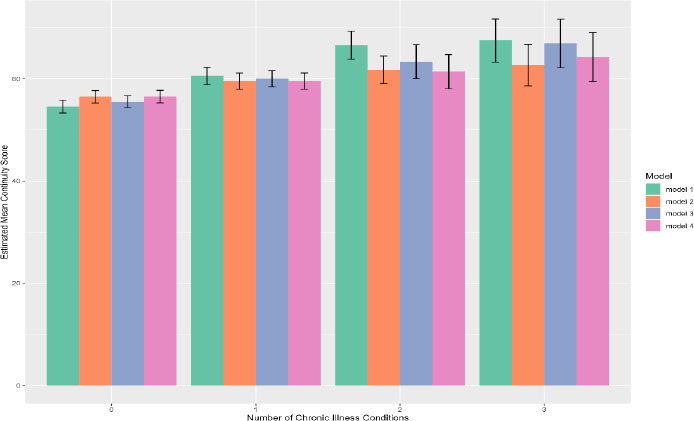
- | Continuity for One Year and More | |||||
---|---|---|---|---|---|---|
Model 3 | Model 4 | |||||
Interaction between Chronic Conditions (No/Yes) and Residency Status (Local/Migrant) | OR* | P | 95%CI** | OR* | P | 95%CI** |
Chronic
Condition: No Residency Status: Migrant and Local |
Ref | Ref | Ref | Ref | Ref | Ref |
Chronic
Condition: Yes Residency Status: Migrant |
0.79 | 0.409 | 0.45, 1.39 | 0.58 | 0.854 | 0.53, 1.69 |
Interaction between Chronic Conditions (No/Low/High) and Residency Status (Local/Migrant) | - | - | - | - | - | - |
Chronic
Condition: No Residency Status: Migrant and Local |
Ref | Ref | Ref | Ref | Ref | Ref |
Chronic
Condition: Low Residency Status: Migrant |
0.81 | 0.512 | 0.43, 1.52 | 0.97 | 0.934 | 0.51, 1.87 |
Chronic
Condition: High Residency Status: Migrant and Local |
0.82 | 0.674 | 0.31, 1.09 | 0.89 | 0.809 | 0.34, 2.31 |
- | Continuity Domain Score | |||||
- | Model 3 | Model 4 | ||||
Interaction between Chronic Conditions (No/Yes) and Residency Status (Local/Migrant) | Coef*** | P | 95% CI | Coef*** | P | 95% CI** |
Chronic
Condition: No Residency Status: Migrant and Local |
Ref | Ref | Ref | Ref | Ref | Ref |
Chronic
Condition: Yes Residency Status: Migrant |
-2.09 | 0.268 | -5.80, 1.61 | 0.19 | 0.000 | -3.47-3.86 |
Interaction between Chronic Conditions (No/Low/High) and Residency Status (Local/Migrant) | - | - | - | - | - | - |
Chronic
Condition: No Residency Status: Migrant and Local |
Ref | Ref | Ref | Ref | Ref | Ref |
Chronic
Condition: Low Residency Status: Migrant |
-1.64 | 0.429 | -5.72, 2.43 | 0.39 | 0.848 | -3.64-4.42 |
Chronic
Condition: High Residency Status: Migrant and Local |
0.63 | 0.850 | -5.88, 7.14 | 1.64 | 0.613 | -4.71-7.99 |
Our study also examined the relationship using a 3-year continuity indicator (k3) and found no significant association between the chronic condition and k3. This implies that long-term continuity is less sensitive to chronic conditions than short-term continuity. In addition, it was also found that the residency status of the patients did not significantly modify the association between chronic conditions and continuity.
Compared to patients without any chronic conditions, patients with chronic conditions had a long relationship with their PCP and perceived a higher continuity score, indicating that they might have more trust in and dependency on their PCP, which, in turn, benefits their health [32]. Also, it was found that a PCP-patient relationship served as a mediating factor in the link between continuity of primary care and chronic conditions. This pattern indicates that having a high number of chronic conditions increases the likelihood of having a primary care contract service, which ultimately increases continuity with the same PCP. Detailed results from the mediation analysis can be found in supplementary materials.
Participants with more than one chronic condition tend to require special attention and specific patient-centered treatment adjustment. Such a treatment plan may require health practitioners to have a thorough understanding of the patient's medical history. Switching primary care practitioners may be inconvenient and inefficient in case of disease detection and follow-up treatment. High use of specialty care may strain clinicians' ability to coordinate care effectively, especially for patients with chronic conditions. This was asserted by an interview-based cross-sectional study conducted on Medicare enrollees with select chronic conditions in an integrated healthcare delivery system in Washington, USA [33] that used encounter data and measured continuity of care for 1 year by applying the Bice-Boxerman measure of continuity [34]. Among high-risk patients with acute and chronic diseases, continuity of care is most important as it will lower the hospitalization cost while enhancing coordinated care and ensuring timely treatment. Patients with chronic disease reported higher continuity, while those who lived in rural areas, had higher education, poorer mental health status, no regular provider, and who were employed reported lower continuity based on a survey data analysis conducted in Ontario, Canada [35], where measurement of continuity was a proportional score calculated from the responses of the participants.
The presence of multiple chronic conditions is linked to an increase in clinic visits (usually outpatients). The relationship between PCP and their patients becomes stronger as the frequency of outpatient visits rises. This long-lasting, reliable relationship makes it possible for PCP to continuously gather patient data, develop a more thorough understanding of patient's needs, make it easier to identify different patient needs, offer comprehensive patient services, and refer patients to more suitable secondary or tertiary care facilities [36-39]. Improved communication between PCP and their patients would encourage the interaction mechanism between continuity of services and other primary care core values. This may reinforce the relationship between providers and patients and provide cumulative benefits for patients as well as lower hospital admission rates.
Another issue highlighted in this study is that we are able to use the continuity parameters proposed by Smedby et al. [40]. Most of the previous studies done in China used their own questionnaires and the score associated with these questionnaires was used as primary outcomes [27, 32, 41]. There is not any universal technique to calculate the continuity scores, which makes it difficult to compare different studies. To measure continuity of care, Smedbly et. al. in their paper [40] proposed visit-based measures, which consider the number of visits within a specifically defined measurement period. The measurement of known provider continuity (k) represents the number of visits within the continuity-determining period (CDP). To be specific, k =1, refers that the primary care provider of the current visit also visited at some other point of time within the continuity determining period, if not, k is considered 0. In this study, we utilized this measure and modified it to consider for continuity of 1 year (k1), where the measurement period (MP) was a 1-year interval before the current visit, and continuity for at least 3 years (k3), where the measurement period (MP) was 3 years before the current visit.
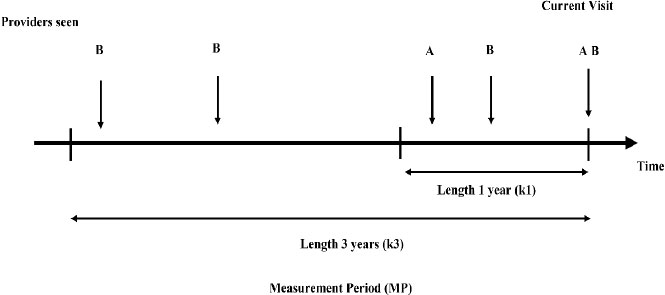
Fig. (3) illustrates how the continuity of care is calculated in this paper. Here, A and B represent the PCP. The horizontal line represents time with an interval of 1 year and 3 years. Here, for provider A, k1 = 0, since A appears among the primary care practitioners seen within less than a year of MP. On the contrary, k1 = 1 for provider B since the length of the continuity was more than 1 year. The survey questionnaire used in this study facilitated the information necessary for using this theory to measure continuity of care. The question utilized in this regard was “How long have you been seeing the primary care physician” with four response options, less than 1 year, 1-3 years, 3-5 years, and more than 5 years. After re-cording these responses to less than a year and more than a year, we obtained a similar outcome variable that measures continuity of 1 year or more with the same primary care practitioner and an outcome variable that measures continuity of 3 years or more with the same PCP.
5. LIMITATIONS
In developing countries like China, obtaining real-time encounter data from electronic health records is difficult. Most of the continuity measurements were obtained from surveys. In this study, we attempted to incorporate quantitative measurements on continuity of care using specific survey questions as a proxy to the encounter data. However, using the proxy may lead to a biased estimate. In addition, this study may be subject to several other biases. First, as this is a cross-sectional study, it will not be appropriate to make causal inferences based on the results. Second, the survey is conducted on data solely based on urban areas in Guangdong province. Thus, this study may lose generalizability. Third, the selection procedure of the survey participants was not randomized so it was a convenient sample. Despite that, the sample size used for the analysis purpose was relatively large with no missing data; this would potentially correct some types of biases mentioned above.
CONCLUSIONS
Our results indicated that the number of chronic conditions is significantly associated with patients' continuity with their PCPs. The odds of visiting the same PCP were higher if the patient suffered from more chronic conditions. The status of residency did not significantly modify this association. Furthermore, the long-term contract between patients and PCPs could moderate the effect of chronic conditions on continuity. Since the relationship between chronic conditions and continuity can reinforce the relationship between providers and patients cumulatively, we recommend policymakers to make policies that facilitate patients with chronic conditions to use primary care services and make a contract with their PCPs.
AUTHORS' CONTRIBUTIONS
N.H. and S.C. designed the study, determined the methodology, and selected the analytical tools. selected the methodology and software; N.H. performed validation; S.C performed the formal analysis. An investigation was done by S.C., M.Z, L.K, and N.H. Data were collected by M,Z, and L.K. Furthermore, S.C. contributed to data curation. S.C. participated in writing – original draft preparation. Writing – reviewing and editing were done by N.H. S.C. contributed to visualization. N.H. contributed to supervision and project administration. All authors have read and agreed to the published version of the manuscript
LIST OF ABBREVIATIONS
(PCPs) | = Primary Care Providers |
(EHRs) | = Electronic Health Records |
(PPS) | = Probability Proportional to Size |
(ASPC) | = Assessment Survey of Primary Care |
(SD) | = Standard Deviation |
ETHICS APPROVAL AND CONSENT TO PARTICIPATE
Ethical approval was obtained from the Institutional Review Board of the School of Public Health, Sun Yat-Sen University, P.R. China (Medical Ethics [2018]014).
HUMAN AND ANIMAL RIGHTS
No Animals were used that are the basis of this study. All human procedures were performed as per the Declaration of Helsinki.
CONSENT FOR PUBLICATION
Informed consent was obtained from all participate.
STANDARDS OF REPORTING
Strengthening the Reporting of Observational Studies in Epidemiology (STROBE) guidelines and methodologies were followed in this study.
FUNDING
None.
AVAILABILITY OF DATA AND MATERIALS
Not applicable.
CONFLICT OF INTEREST
The authors declare that they have no competing interests.
ACKNOWLEDGEMENTS
The authors would like to thank Justin Hu for his help with language editing. The authors would also like to thank all reviewers for their insightful reviews and recommendations.
SUPPLEMENTARY MATERIALS
Supplementary material is available on the Publisher’s website.