Deployment of a Phenotypic Characterization System for Effective Identification of the Onset of Asthma Disease
Abstract
Background
Essentially, machine learning techniques help with clinical decision-making by forecasting prediction results based on recent and historical data, which are frequently found in carefully chosen clinical data repositories. In order to uncover hidden patterns in the data, machine learning applies sophisticated analytical techniques that conduct an exploratory analysis while constructing prediction models to support clinical judgment.
Objective
To effectively identify asthmatics in two distinct cohorts representing India's rural and urban populations by adopting a phenotypic characterization approach.
Methods
Cross-sectional and categorical in design, the data represent the two populations, with clinical history information emphasizing clinical symptoms and patterns defining the condition. The method adopts a hybrid approach since it uniquely blends the unsupervised and supervised learning techniques to explore the advantages of both. The clustering data emphasizing the phenotypic characteristics of asthma is input to the classifier, and the performance of the classifier was continuously monitored for significant improvement in the results.
Results
Asthma disease outcome predictions made by the hybrid decision support system were quite accurate, with classification accuracy reaching up to 85.1% and 95.3% for the two datasets, respectively.
Conclusion
Since asthma is a heterogeneous disease with multiple subtypes, employing clustering information in the form of cluster evaluation scores as an input parameter to the classifiers can effectively predict disease outcomes.
1. INTRODUCTION
Asthma is one of the most common long-term pulmonary respiratory disorders. Itis recognized to be influenced by several variables, including genetics and environmental elements that are crucial to the disease's persistence and advancement [1]. When evaluated using data from electronic health records, clinical symptoms, their patterns, and their frequency significantly influence the course and impact of the disease [2]. The well-known ISAAC developed core questionnaires on asthma and associated comorbidities, which have been well-received in most pilot studies in various countries. It has been suggested that a two-stage hybrid decision support system is created, with the first stage's outputs serving as a major input. [3-5]. Our study has a solid chance of serving as a significant intervention by facilitating early risk assessment and efficient illness prognosis through risk stratification. The disease may grow and ultimately become irreversible if it is not detected at an early stage, even though it is reversible in nature.
Clinicians and clinical researchers frequently use predictive models to identify and assess asthmatic patients' risks and weigh the advantages of preventive interventions against their costs and inconveniences [6, 7]. We present a weighted feature averaging technique for extracting the most relevant and prominent features concerning asthma outcome—the problem under the study's target.
Without any regard to the underlying illness, a phenotype refers to the distinguishable features of a disease, such as its clinical, morphologic, physiological, biochemical, and therapeutic response [8]. An individual's phenotype, which can change over time as a result of interactions between the environment and genes, includes having asthma. Classifying asthma can be challenging because there can be significant similarities between phenotypes. The best strategy to achieve better results would be to stratify asthma based on phenotypes or endotypes [9-11]. Below are other similar studies, and it has been widely observed and deduced that unsupervised learning utilizing cluster analysis has been one of the strategies used to undertake asthma phenotyping.
Predictive models and decision support systems currently in use perform poorly and with insufficient precision. There are ongoing efforts to enhance the same, and one such effort in this area was made by us when we adopted a system that effectively combined clustering and classification learning approaches to get the best prediction accuracies at an early stage [12]. An empirical investigation of the various standard learning classifiers described previously shows that the suggested solution outperforms the traditional classifiers for predicting the course of the disease. The system, therefore created, has a good sensitivity-to-specificity ratio, indicating that one may trust the constructed model to accurately forecast the condition. It can be incorporated into any asthma tools that provide self-management surveys by doing this.
By utilizing both unsupervised and supervised techniques, the decision support system's main goal is to accurately predict the course of the asthma illness. The system works in two steps: first, a target feature cluster is identified, which then determines the relevant features using a modified version of fuzzy C Means clustering; second, subject clusters are formed, with samples within each cluster sharing a common phenotype. Forecasting of illness outcome is achieved by adjusting a binary classification tree on the given data, which now includes categorical data indicative of identified subtypes of asthma created from the preliminary stage involving subject clustering.
The approach has successfully classified many asthma phenotypic groups with associated clinical risk factors, which is a crucial step in promoting the use of the appropriate medications early in the course of the disease to lessen its damaging effects. However, the clinical results in this work were mostly calculated based only on medical history.
1.1. Related Work
To explore asthma data and uncover latent patterns that add value, clinical decision support systems that use machine learning techniques to mine the useful data may be implemented. This would offer an additional source of greater understanding for decision-making regarding the identification of risk factors and the assessment of the disease's severity [13-15]. Also, it would be helpful to evaluate the degree to which it relates to other lung diseases like Chronic Obstructive Pulmonary Disease (COPD). [16-18].
Predictive models and decision assistance systems currently in use perform poorly with poor precision. As part of our continued efforts to improve the system, we adopted a method that successfully combined clustering and classification learning approaches to achieve the best prediction accuracies. An empirical investigation of the various standard learning classifiers described in the previous studies shows that the suggested solution outperforms the traditional classifiers for predicting the course of the disease. The system, therefore, has a good sensitivity-to-specificity ratio, indicating that one may trust the constructed model to accurately forecast the condition. This allows it to be incorporated into all asthma tools that provide self-management questionnaires. Incorporating supervised learning techniques into Bayesian classification approaches has produced classes that are primarily recognized as correct and have a good amount of face validity, as well as significant relationships to asthma, variations in airway reactivity, and lung function, which demonstrate content validity and provide a good amount of satisfaction [19-21]. In order to assess the risk factors, predictive analytics havebeen effectively used to combine independent data from varied sources [22]. The population of asthmatics who attend emergency rooms is described by rule-based systems that integrate expert-driven feature selection with rough sets techniques [23]. Depending on the underlying data type and the ensemble learning strategies used, a respectable level of precision was achieved in the job of distinguishing asthma from other respiratory illnesses. [24,25,21,26].
The work is centered on outlining and showcasing the efficient application of machine learning methods to support clinical judgments about the early identification and evaluation of asthma, a long-term respiratory condition that affects the respiratory system. The usefulness of clinical data-driven predictive models using machine learning for asthma prognosis due to efforts to enhance clinical procedures and attain better disease outcomes, history, and findings has been steadily increasing [27]. This can be very advantageous for doctors and patients, thus cutting down on overall expenses.
Disease predictive modeling is carried out by utilizing instruments that are thought to aid in decision-making. While some concentrate on forecasting a clinical outcome, others categorize patients who might be susceptible to forming a specific medical condition. Including domain expertise in finding the clinical characteristics that are most pertinent to prediction models entails disease classification based on a prior understanding of the illness. Although physicians still employ a subjective method, machine learning techniques for predictive modeling of healthcare and medical data have become more popular recently. To uncover hidden relationships in clinical data, machine learning techniques primarily use data-driven approaches to explore the nature of the data. They then examine the data to identify intriguing trends that lead to a useful prognosis for a particular illness.
When it comes to providing the best level of precision, traditional machine learning techniques have their own limitations. The reasons for this include the presence of noise or outliers in the input data, the inability to normalize the data, the existence of redundant and irrelevant attributes, the lack of diversity in the training data, and the use of incorrect estimation techniques to determine the machine learning methods' accuracy level. Therefore, unless machine learning techniques are specifically designed to handle unknown data, using them as a solution is not a good idea. Instead, optimization techniques should be used.
2. MATERIAL AND METHODS
2.1. Dataset
It is observed that the current asthma prediction models have lower accuracy levels and are not trusted by medical professionals, thus, attempts are being made to make improvements [28]. Various subjective evaluation methods for clinical evaluation of asthma, include the score on the symptom tracker and the asthma control questionnaire. Recently, questionnaires have been used to gather firsthand information on the Probability of subjects having asthma at risk. The demographic and environ- mental information, the frequency and incidence of prognostic factors like wheezing episodes, parental history of the disease, comorbidities like hay fever and rhinitis, and symptoms like cough, wheezing, allergies, eczema, sleep and speech disturbances and their varied presentations are all shared among these questionnaires. Since there is no gold standard to characterize disease patterns, it is possible that different questionnaire data have some variation in the factors considered for the study of the disease. Therefore, we turn to the ISAAC data, which is standard clinical asthma questionnaire data that has been accepted and used in several pilot studies around the world.
The system performance was validated using response data from the ISAAC core questionnaires collected during the first phase of the study, which has been applied to multiple pilot studies [29]. Usually, the data collection questions represent delicate and particular disease indicators. The data are cross-sectional because they are analyzed with respect to a specific population, which is typically a sizable subset that is most representative at a given moment in time. Two age groups were used to collect the data: 6–7 years and 13–14 years. While the older children in one group completed the response indepen- dently, the parents in the other group completed the same task. The New Delhi dataset included information on 2961 individuals that was analyzed in relation to 47 variables that were suggestive of symptoms and comorbidities related to asthma. For 112 samples, the disease outcome was reported as unknown. Therefore, we eliminated all 112 samples, resulting in a dataset with 2849 samples. Since there were only 110 asthmatic subjects in the entire population, we attempted to address this imbalance by using stratified sampling, which allowed us to select 110 samples from the non-asthmatic group as well. This allowed us to create a balanced dataset, fed as the input data. Similarly, in order to counteract the class imbalance that would have produced 78 asthmatics, we attempted to select 2% of the total population, or 64 subjects, from the non-asthmatic group.
2.2. Clustering of Features and Phenotypic Characterization
Feature clustering is first used to select the most common features that can be recognized as important comorbidities of asthma disease. Unlike the traditional Fuzzy C Means technique, which uses an objective function based on distances, Modified Fuzzy C Means Clustering (MFCM) uses an objective function based on correlation to cluster features. Based on subtractive clustering evaluation results, we decided to divide the input feature set into four clusters. The cluster containing the asthma attribute extracts the features that coexist with the asthma attribute. As a result, the process's feature set is diminished.
Fuzzy C Mean Clustering uses an objective function that is based on correlation. Here, the distance metric includes a Pearson correlation coefficient, which shows how different two features are from one another or from a cluster. A positive or negative correlation can be indicated by the direct or inverse correlation between features. All related features will be grouped together regardless of how they are expressed. The definition of the distance metrics is dij = 1− ρ2 Xi, C j, where ρXi, C j is the Pearson measure of the correlation between a feature (xi) and a cluster (cj). In the event of a high correlation—positive or negative—the correlation would be 0.
To generate silhouette scores, we employed K means clustering, and the number of clusters used to indicate the “K” parameter was adjusted from 2 to 8. With the naive data, the silhouette score was high for two clusters, and with the New Delhi data, it was high for eight clusters. In Fig. (1a and b), the distribution patterns of asthmatics and non-asthmatics in the various clusters are clearly shown. With the decreased feature set, the participants in the two datasets were grouped into two and eight clusters, respectively. After that, a classification and regression tree was used to predict people with and without asthma.
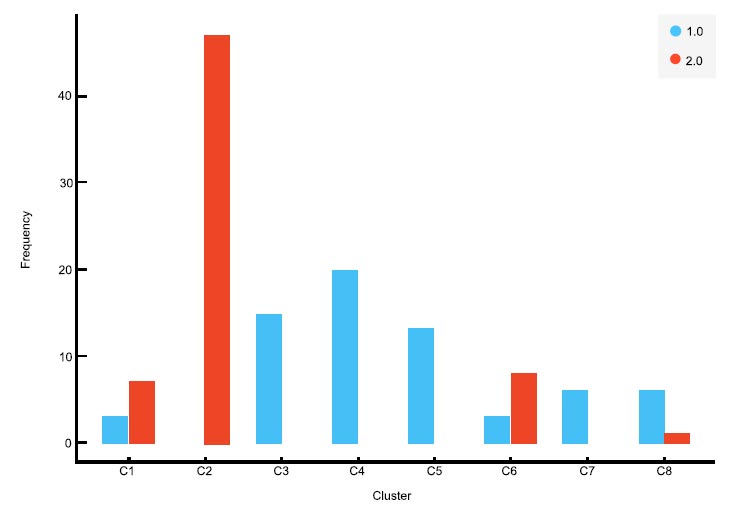
As shown in Tables 1 and 2, both random initialization and K means ++ are used to initialize the cluster centroids for K-means clustering in order to establish a strong basis for the number of clusters chosen. Both initialization methods produced the same result, indicating agreement on selecting two and eight clusters in Naively and New Delhi data, respectively. The silhouette score for Naively data was 0.325 using both methods, and silhouette scores for New Delhi data were 0.384 and 0.377 (highest) using random initialization and K-means++, respectively. The hybrid decision support system predicts the course of an illness.
Initialization Method |
Clusters | Silhouette Score |
---|---|---|
Dataset | Naively data | |
2 | 0.325 | |
Random Initialization |
3 | 0.236 |
4 | 0.217 | |
5 | 0.220 | |
6 | 0.194 | |
7 | 0.204 | |
8 | 0.217 | |
K-Means++ | 2 | 0.325 |
3 | 0.236 | |
4 | 0.217 | |
5 | 0.216 | |
6 | 0.206 | |
7 | 0.219 | |
8 | 0.232 |
Dataset | New Delhi Data | |
---|---|---|
Random initialization |
2 | 0.384 |
3 | 0.376 | |
4 | 0.409 | |
5 | 0.422 | |
6 | 0.442 | |
7 | 0.441 | |
8 | 0.464 | |
K-Means++ | 2 | 0.384 |
3 | 0.377 | |
4 | 0.405 | |
5 | 0.414 | |
6 | 0.446 | |
7 | 0.452 | |
8 | 0.461 |
Dataset | Method | AUC | CA | F1 | Precision | Recall |
---|---|---|---|---|---|---|
Rural | 10-fold Cross Validation | 0.855 | 0.851 | 0.851 | 0.854 | 0.851 |
Urban | 0.977 | 0.953 | 0.953 | 0.954 | 0.953 |
Predicting the course of an illness is the hybrid decision support system's main goal. By utilizing both supervised and unsupervised techniques, asthma with a high degree of accuracy. The system is introduced in two phases, the first of which is the Target feature cluster identification, which leads to the identification of the pertinent features utilizing a modified alternative to Fuzzy C means clustering, then a subsequent stage when subject clusters are formed such that each is characterized by samples that share a common phenotype. The forecast of Fitting a binary classification tree to the input data, which now includes the illness outcome, provides category information indicating the previously known subgroups of asthma and grouping of subject-related stages.
3. RESULTS
Table 3 illustrates the performance of the hybrid decision system as determined by 10-fold cross-validation, stratified random sampling, and random sampling. It shows an analysis of the performance of the classifiers in comparison to other classifiers. It has been noted that the hybrid decision system outperforms the other classifiers in terms of performance.
4. DISCUSSION
Two testing schemes were used to assess the performance: 10-fold cross-validation and stratified random sampling, which are covered in more detail below.
By dividing the initial sample into a training set for model training and a test set for performance evaluation, the cross-validation technique is used to assess prediction models. The provided input sample is thoroughly divided into ten equal-size subsamples for 10-fold cross-validation. Nine of the remaining “k” subsamples are used to train the model, with one sample set aside as part of the validation set for model testing. There are ten iterations in the process. One estimate can then be generated by averaging the outcomes of these ten folds. Stratified random sampling is.
In situations where the strata are assumed to be too dissimilar and too important to the problem under study and/or when investigators want to oversample a primarily minor group of interest, the chosen technique is used instead of simple random sampling. In this case, the entire dataset is divided into train and test sets.
To confirm that the findings are accurate, the procedure is repeated 100 times using 20% of the samples in the test set and 80% of the samples in the train set. According to a performance comparison between conventional classifiers and the optimized gradient boosting method, the latter method offers a higher classification accuracy, with 10-fold cross-validation yielding results of 84.5% and 91.4% for the Neyveli and New Delhi datasets, respectively.
The proposed method is seen to perform better than the conventional classifiers for an empirical investigation of the numerous traditional learning classifiers shown in the previous studies revealed the prediction of disease outcome. Thus, the system was created to ensure a strong sensitivity to specificity ratio, indicating that the model created to forecast the illness can be successfully deployed effectively. This allows it to be incorporated into any asthma tools thatprovides self-management tests or any other resources the therapist offers that are aimed at preliminary disease detection screening.
CONCLUSION
The system proposed and developed has a good sensitivity to specificity ratio, indicating that one may trust the constructed model to accurately forecast the condition. This allows it to be incorporated into all asthma tools that provide self-management questionnaires. The performance evaluation clearly shows that the developed hybrid decision support system explores the advantages of both supervised and unsupervised learning techniques, and results obtained are quite promising for appropriate deployment in real-time scenarios. For the two datasets we examined in our study, the hybrid decision support system achieved classification accuracy as high as 85.1% and 95.3%, demonstrating its effectiveness in making accurate predictions regarding the outcome of asthma disease. Additionally, the two datasets’ respective precision rates of 85.4% and 95.4% were satisfactory. Furthermore, with asthma being considered a heterogeneous disease with a wide range of symptoms, using clustering information as an input parameter to classifiers in the form of cluster evaluation scores can effectively predict disease outcomes.